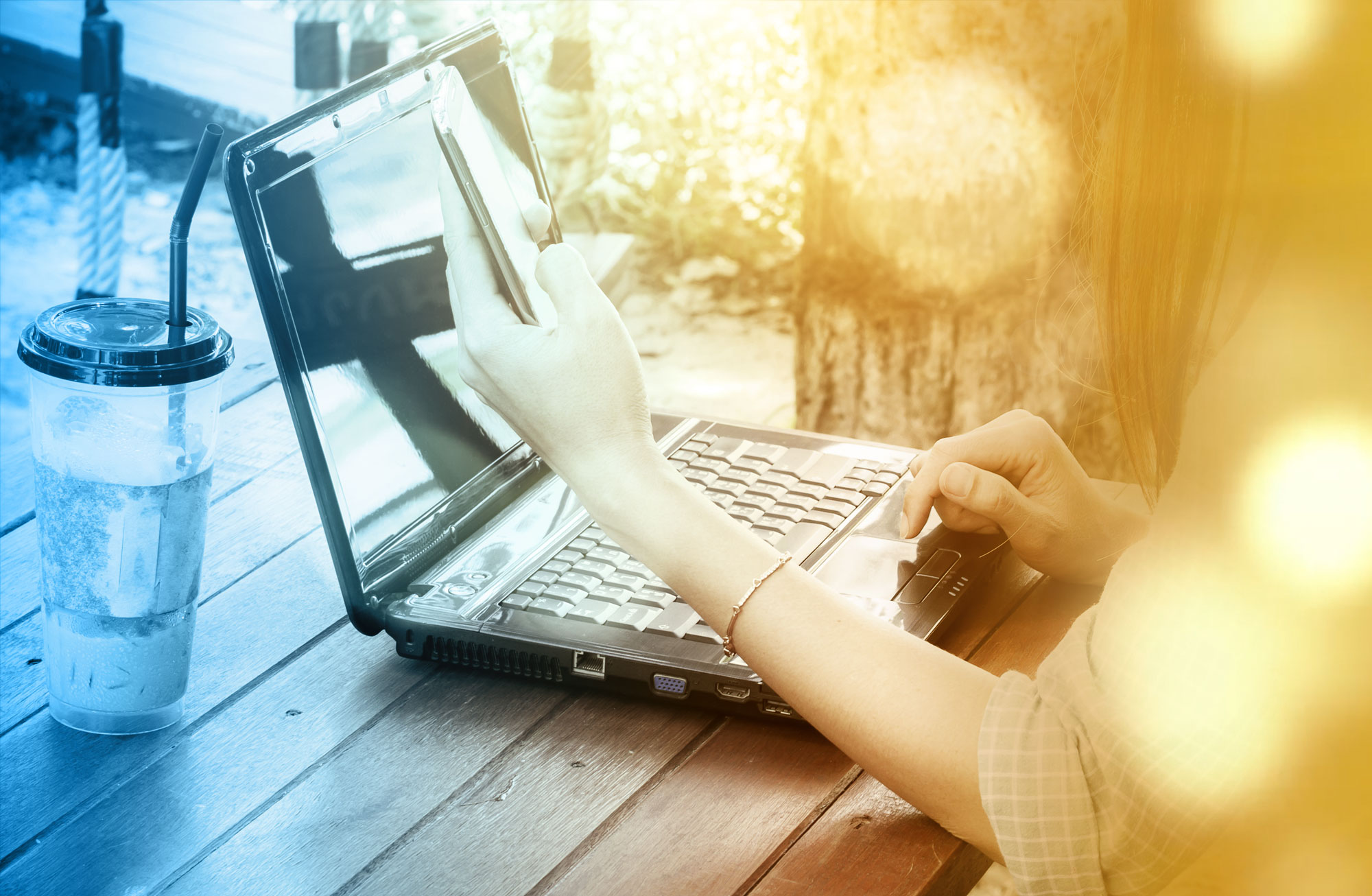
Table of Contents
Analyzing the Real-World Applications and Value of AI for eDiscovery
By Karl Sobylak, Director of Product Management, AI, Lighthouse; Fernando Delgado, Director of AI Analytics, Lighthouse;Sarah Moran, eDiscovery and AI Evangelist, Lighthouse
Advancements in artificial intelligence (AI) are raising questions and opportunities in every industry. AI capabilities like natural language processing, prediction, and content generation have taken massive leaps forward in recent years, leading to headline-grabbing platforms like ChatGPT, as well as other tools that receive less attention but are no less impactful.
In the legal profession, advanced AI is already changing how firms and in-house counsel approach eDiscovery. The scale of and intricacy of document review, in particular, makes it an ideal domain for the computational power and nuanced analysis that AI is capable of today.
This whitepaper provides legal teams with practical information about how advanced AI can support their document review efforts. This includes straightforward explanations and real-world examples for using AI on single matters, multiple matters, and at the portfolio level.
Advancements in AI are only going to continue, so it’s important to get familiar with them now. We hope this paper brings the new frontier of AI a little closer to home so that teams can make the most informed and effective plans for document review.
AI for Single Matters: Refining Responsiveness
What It Is
Advanced AI builds responsive sets that are significantly smaller and more precise than those built by classic TAR models.
The most common technology-assisted review (TAR) models used in eDiscovery were designed in the 1970s, so their capacity is limited. They recognize words but not context, they’re blind to metadata, and in general they search and analyze documents at a superficial level. As a result, classic TAR models create large responsive sets with many documents that aren’t actually relevant, while leaving out many documents that are.
Advanced AI takes a much more comprehensive look, which makes it much more precise at capturing relevant documents and discarding irrelevant ones. It uses multiple layers of learning networks to incorporate a higher volume of data and broader range of data types and sources into its algorithms. It also utilizes natural language processing (NLP), which studies words in context to get a nuanced interpretation of meaning. This makes NLP much more accurate than older models when classifying responsiveness, privilege, and other categories.
Why It’s Valuable
Advanced AI enables legal teams to avoid the excessive delays and cost of reviewing thousands of irrelevant documents.
The benefits of a smaller and more precise responsive set are obvious: With fewer documents for eyes-on review, the whole effort is faster and less expensive.
And the need for these benefits becomes more pronounced every day. Datasets are swelling to millions of documents, while legal budgets are shrinking. Legal teams must reduce the responsive set in a cost-efficient and defensible way.
The cost of using advanced AI analytics is often outweighed by the savings it enables. TAR workflows that incorporate advanced AI have been approved by multiple courts and U.S. regulatory agencies for various matters, and a good eDiscovery partner will advise you on projected ROI and how the technology works so you can represent it with authority during litigation.
In the Real World
Advanced AI outperformed traditional TAR models during an HSR Second Request, using the same data and parameters.
During a recent Hart-Scott-Rodino Second Request, outside counsel for a Fortune 500 technology company used advanced AI to create a responsive set of documents for submission to the Department of Justice. The firm also ran two popular TAR models on the same data, using the same control set, to compare performance and inform the firm’s approach to future matters.
Advanced AI outperformed traditional TAR in several critical metrics:
- 430 fewer documents found to be potentially responsive than. the nearest alternative
- 50% fewer documents for privilege review, compared to classical TAR model
- 70% fewer foreign-language documents requiring translation and review, compared to one TAR model
- Overall savings of 18K review hours and $2M
AI for Single Matters: Refining Responsiveness
What It Is
Advanced AI builds responsive sets that are significantly smaller and more precise than those built by classic TAR models.
The most common technology-assisted review (TAR) models used in eDiscovery were designed in the 1970s, so their capacity is limited. They recognize words but not context, they’re blind to metadata, and in general they search and analyze documents at a superficial level. As a result, classic TAR models create large responsive sets with many documents that aren’t actually relevant, while leaving out many documents that are.
Advanced AI takes a much more comprehensive look, which makes it much more precise at capturing relevant documents and discarding irrelevant ones. It uses multiple layers of learning networks to incorporate a higher volume of data and broader range of data types and sources into its algorithms. It also utilizes natural language processing (NLP), which studies words in context to get a nuanced interpretation of meaning. This makes NLP much more accurate than older models when classifying responsiveness, privilege, and other categories.
Why It’s Valuable
Advanced AI enables legal teams to avoid the excessive delays and cost of reviewing thousands of irrelevant documents.
The benefits of a smaller and more precise responsive set are obvious: With fewer documents for eyes-on review, the whole effort is faster and less expensive.
And the need for these benefits becomes more pronounced every day. Datasets are swelling to millions of documents, while legal budgets are shrinking. Legal teams must reduce the responsive set in a cost-efficient and defensible way.
The cost of using advanced AI analytics is often outweighed by the savings it enables. TAR workflows that incorporate advanced AI have been approved by multiple courts and U.S. regulatory agencies for various matters, and a good eDiscovery partner will advise you on projected ROI and how the technology works so you can represent it with authority during litigation.
In the Real World
Advanced AI outperformed traditional TAR models during an HSR Second Request, using the same data and parameters.
During a recent Hart-Scott-Rodino Second Request, outside counsel for a Fortune 500 technology company used advanced AI to create a responsive set of documents for submission to the Department of Justice. The firm also ran two popular TAR models on the same data, using the same control set, to compare performance and inform the firm’s approach to future matters.
Advanced AI outperformed traditional TAR in several critical metrics:
- 430 fewer documents found to be potentially responsive than. the nearest alternative
- 50% fewer documents for privilege review, compared to classical TAR model
- 70% fewer foreign-language documents requiring translation and review, compared to one TAR model
- Overall savings of 18K review hours and $2M
AI for Single Matters: Refining Responsiveness
What It Is
Advanced AI builds responsive sets that are significantly smaller and more precise than those built by classic TAR models.
The most common technology-assisted review (TAR) models used in eDiscovery were designed in the 1970s, so their capacity is limited. They recognize words but not context, they’re blind to metadata, and in general they search and analyze documents at a superficial level. As a result, classic TAR models create large responsive sets with many documents that aren’t actually relevant, while leaving out many documents that are.
Advanced AI takes a much more comprehensive look, which makes it much more precise at capturing relevant documents and discarding irrelevant ones. It uses multiple layers of learning networks to incorporate a higher volume of data and broader range of data types and sources into its algorithms. It also utilizes natural language processing (NLP), which studies words in context to get a nuanced interpretation of meaning. This makes NLP much more accurate than older models when classifying responsiveness, privilege, and other categories.
Why It’s Valuable
Advanced AI enables legal teams to avoid the excessive delays and cost of reviewing thousands of irrelevant documents.
The benefits of a smaller and more precise responsive set are obvious: With fewer documents for eyes-on review, the whole effort is faster and less expensive.
And the need for these benefits becomes more pronounced every day. Datasets are swelling to millions of documents, while legal budgets are shrinking. Legal teams must reduce the responsive set in a cost-efficient and defensible way.
The cost of using advanced AI analytics is often outweighed by the savings it enables. TAR workflows that incorporate advanced AI have been approved by multiple courts and U.S. regulatory agencies for various matters, and a good eDiscovery partner will advise you on projected ROI and how the technology works so you can represent it with authority during litigation.
In the Real World
Advanced AI outperformed traditional TAR models during an HSR Second Request, using the same data and parameters.
During a recent Hart-Scott-Rodino Second Request, outside counsel for a Fortune 500 technology company used advanced AI to create a responsive set of documents for submission to the Department of Justice. The firm also ran two popular TAR models on the same data, using the same control set, to compare performance and inform the firm’s approach to future matters.
Advanced AI outperformed traditional TAR in several critical metrics:
- 430 fewer documents found to be potentially responsive than. the nearest alternative
- 50% fewer documents for privilege review, compared to classical TAR model
- 70% fewer foreign-language documents requiring translation and review, compared to one TAR model
- Overall savings of 18K review hours and $2M
AI for Single Matters: Refining Responsiveness
What It Is
Advanced AI builds responsive sets that are significantly smaller and more precise than those built by classic TAR models.
The most common technology-assisted review (TAR) models used in eDiscovery were designed in the 1970s, so their capacity is limited. They recognize words but not context, they’re blind to metadata, and in general they search and analyze documents at a superficial level. As a result, classic TAR models create large responsive sets with many documents that aren’t actually relevant, while leaving out many documents that are.
Advanced AI takes a much more comprehensive look, which makes it much more precise at capturing relevant documents and discarding irrelevant ones. It uses multiple layers of learning networks to incorporate a higher volume of data and broader range of data types and sources into its algorithms. It also utilizes natural language processing (NLP), which studies words in context to get a nuanced interpretation of meaning. This makes NLP much more accurate than older models when classifying responsiveness, privilege, and other categories.
Why It’s Valuable
Advanced AI enables legal teams to avoid the excessive delays and cost of reviewing thousands of irrelevant documents.
The benefits of a smaller and more precise responsive set are obvious: With fewer documents for eyes-on review, the whole effort is faster and less expensive.
And the need for these benefits becomes more pronounced every day. Datasets are swelling to millions of documents, while legal budgets are shrinking. Legal teams must reduce the responsive set in a cost-efficient and defensible way.
The cost of using advanced AI analytics is often outweighed by the savings it enables. TAR workflows that incorporate advanced AI have been approved by multiple courts and U.S. regulatory agencies for various matters, and a good eDiscovery partner will advise you on projected ROI and how the technology works so you can represent it with authority during litigation.
In the Real World
Advanced AI outperformed traditional TAR models during an HSR Second Request, using the same data and parameters.
During a recent Hart-Scott-Rodino Second Request, outside counsel for a Fortune 500 technology company used advanced AI to create a responsive set of documents for submission to the Department of Justice. The firm also ran two popular TAR models on the same data, using the same control set, to compare performance and inform the firm’s approach to future matters.
Advanced AI outperformed traditional TAR in several critical metrics:
- 430 fewer documents found to be potentially responsive than. the nearest alternative
- 50% fewer documents for privilege review, compared to classical TAR model
- 70% fewer foreign-language documents requiring translation and review, compared to one TAR model
- Overall savings of 18K review hours and $2M
AI for Single Matters: Refining Responsiveness
What It Is
Advanced AI builds responsive sets that are significantly smaller and more precise than those built by classic TAR models.
The most common technology-assisted review (TAR) models used in eDiscovery were designed in the 1970s, so their capacity is limited. They recognize words but not context, they’re blind to metadata, and in general they search and analyze documents at a superficial level. As a result, classic TAR models create large responsive sets with many documents that aren’t actually relevant, while leaving out many documents that are.
Advanced AI takes a much more comprehensive look, which makes it much more precise at capturing relevant documents and discarding irrelevant ones. It uses multiple layers of learning networks to incorporate a higher volume of data and broader range of data types and sources into its algorithms. It also utilizes natural language processing (NLP), which studies words in context to get a nuanced interpretation of meaning. This makes NLP much more accurate than older models when classifying responsiveness, privilege, and other categories.
Why It’s Valuable
Advanced AI enables legal teams to avoid the excessive delays and cost of reviewing thousands of irrelevant documents.
The benefits of a smaller and more precise responsive set are obvious: With fewer documents for eyes-on review, the whole effort is faster and less expensive.
And the need for these benefits becomes more pronounced every day. Datasets are swelling to millions of documents, while legal budgets are shrinking. Legal teams must reduce the responsive set in a cost-efficient and defensible way.
The cost of using advanced AI analytics is often outweighed by the savings it enables. TAR workflows that incorporate advanced AI have been approved by multiple courts and U.S. regulatory agencies for various matters, and a good eDiscovery partner will advise you on projected ROI and how the technology works so you can represent it with authority during litigation.
In the Real World
Advanced AI outperformed traditional TAR models during an HSR Second Request, using the same data and parameters.
During a recent Hart-Scott-Rodino Second Request, outside counsel for a Fortune 500 technology company used advanced AI to create a responsive set of documents for submission to the Department of Justice. The firm also ran two popular TAR models on the same data, using the same control set, to compare performance and inform the firm’s approach to future matters.
Advanced AI outperformed traditional TAR in several critical metrics:
- 430 fewer documents found to be potentially responsive than. the nearest alternative
- 50% fewer documents for privilege review, compared to classical TAR model
- 70% fewer foreign-language documents requiring translation and review, compared to one TAR model
- Overall savings of 18K review hours and $2M
AI for Single Matters: Refining Responsiveness
What It Is
Advanced AI builds responsive sets that are significantly smaller and more precise than those built by classic TAR models.
The most common technology-assisted review (TAR) models used in eDiscovery were designed in the 1970s, so their capacity is limited. They recognize words but not context, they’re blind to metadata, and in general they search and analyze documents at a superficial level. As a result, classic TAR models create large responsive sets with many documents that aren’t actually relevant, while leaving out many documents that are.
Advanced AI takes a much more comprehensive look, which makes it much more precise at capturing relevant documents and discarding irrelevant ones. It uses multiple layers of learning networks to incorporate a higher volume of data and broader range of data types and sources into its algorithms. It also utilizes natural language processing (NLP), which studies words in context to get a nuanced interpretation of meaning. This makes NLP much more accurate than older models when classifying responsiveness, privilege, and other categories.
Why It’s Valuable
Advanced AI enables legal teams to avoid the excessive delays and cost of reviewing thousands of irrelevant documents.
The benefits of a smaller and more precise responsive set are obvious: With fewer documents for eyes-on review, the whole effort is faster and less expensive.
And the need for these benefits becomes more pronounced every day. Datasets are swelling to millions of documents, while legal budgets are shrinking. Legal teams must reduce the responsive set in a cost-efficient and defensible way.
The cost of using advanced AI analytics is often outweighed by the savings it enables. TAR workflows that incorporate advanced AI have been approved by multiple courts and U.S. regulatory agencies for various matters, and a good eDiscovery partner will advise you on projected ROI and how the technology works so you can represent it with authority during litigation.
In the Real World
Advanced AI outperformed traditional TAR models during an HSR Second Request, using the same data and parameters.
During a recent Hart-Scott-Rodino Second Request, outside counsel for a Fortune 500 technology company used advanced AI to create a responsive set of documents for submission to the Department of Justice. The firm also ran two popular TAR models on the same data, using the same control set, to compare performance and inform the firm’s approach to future matters.
Advanced AI outperformed traditional TAR in several critical metrics:
- 430 fewer documents found to be potentially responsive than. the nearest alternative
- 50% fewer documents for privilege review, compared to classical TAR model
- 70% fewer foreign-language documents requiring translation and review, compared to one TAR model
- Overall savings of 18K review hours and $2M
AI for Single Matters: Refining Responsiveness
What It Is
Advanced AI builds responsive sets that are significantly smaller and more precise than those built by classic TAR models.
The most common technology-assisted review (TAR) models used in eDiscovery were designed in the 1970s, so their capacity is limited. They recognize words but not context, they’re blind to metadata, and in general they search and analyze documents at a superficial level. As a result, classic TAR models create large responsive sets with many documents that aren’t actually relevant, while leaving out many documents that are.
Advanced AI takes a much more comprehensive look, which makes it much more precise at capturing relevant documents and discarding irrelevant ones. It uses multiple layers of learning networks to incorporate a higher volume of data and broader range of data types and sources into its algorithms. It also utilizes natural language processing (NLP), which studies words in context to get a nuanced interpretation of meaning. This makes NLP much more accurate than older models when classifying responsiveness, privilege, and other categories.
Why It’s Valuable
Advanced AI enables legal teams to avoid the excessive delays and cost of reviewing thousands of irrelevant documents.
The benefits of a smaller and more precise responsive set are obvious: With fewer documents for eyes-on review, the whole effort is faster and less expensive.
And the need for these benefits becomes more pronounced every day. Datasets are swelling to millions of documents, while legal budgets are shrinking. Legal teams must reduce the responsive set in a cost-efficient and defensible way.
The cost of using advanced AI analytics is often outweighed by the savings it enables. TAR workflows that incorporate advanced AI have been approved by multiple courts and U.S. regulatory agencies for various matters, and a good eDiscovery partner will advise you on projected ROI and how the technology works so you can represent it with authority during litigation.
In the Real World
Advanced AI outperformed traditional TAR models during an HSR Second Request, using the same data and parameters.
During a recent Hart-Scott-Rodino Second Request, outside counsel for a Fortune 500 technology company used advanced AI to create a responsive set of documents for submission to the Department of Justice. The firm also ran two popular TAR models on the same data, using the same control set, to compare performance and inform the firm’s approach to future matters.
Advanced AI outperformed traditional TAR in several critical metrics:
- 430 fewer documents found to be potentially responsive than. the nearest alternative
- 50% fewer documents for privilege review, compared to classical TAR model
- 70% fewer foreign-language documents requiring translation and review, compared to one TAR model
- Overall savings of 18K review hours and $2M
AI for Single Matters: Refining Responsiveness
What It Is
Advanced AI builds responsive sets that are significantly smaller and more precise than those built by classic TAR models.
The most common technology-assisted review (TAR) models used in eDiscovery were designed in the 1970s, so their capacity is limited. They recognize words but not context, they’re blind to metadata, and in general they search and analyze documents at a superficial level. As a result, classic TAR models create large responsive sets with many documents that aren’t actually relevant, while leaving out many documents that are.
Advanced AI takes a much more comprehensive look, which makes it much more precise at capturing relevant documents and discarding irrelevant ones. It uses multiple layers of learning networks to incorporate a higher volume of data and broader range of data types and sources into its algorithms. It also utilizes natural language processing (NLP), which studies words in context to get a nuanced interpretation of meaning. This makes NLP much more accurate than older models when classifying responsiveness, privilege, and other categories.
Why It’s Valuable
Advanced AI enables legal teams to avoid the excessive delays and cost of reviewing thousands of irrelevant documents.
The benefits of a smaller and more precise responsive set are obvious: With fewer documents for eyes-on review, the whole effort is faster and less expensive.
And the need for these benefits becomes more pronounced every day. Datasets are swelling to millions of documents, while legal budgets are shrinking. Legal teams must reduce the responsive set in a cost-efficient and defensible way.
The cost of using advanced AI analytics is often outweighed by the savings it enables. TAR workflows that incorporate advanced AI have been approved by multiple courts and U.S. regulatory agencies for various matters, and a good eDiscovery partner will advise you on projected ROI and how the technology works so you can represent it with authority during litigation.
In the Real World
Advanced AI outperformed traditional TAR models during an HSR Second Request, using the same data and parameters.
During a recent Hart-Scott-Rodino Second Request, outside counsel for a Fortune 500 technology company used advanced AI to create a responsive set of documents for submission to the Department of Justice. The firm also ran two popular TAR models on the same data, using the same control set, to compare performance and inform the firm’s approach to future matters.
Advanced AI outperformed traditional TAR in several critical metrics:
- 430 fewer documents found to be potentially responsive than. the nearest alternative
- 50% fewer documents for privilege review, compared to classical TAR model
- 70% fewer foreign-language documents requiring translation and review, compared to one TAR model
- Overall savings of 18K review hours and $2M
AI for Single Matters: Refining Responsiveness
What It Is
Advanced AI builds responsive sets that are significantly smaller and more precise than those built by classic TAR models.
The most common technology-assisted review (TAR) models used in eDiscovery were designed in the 1970s, so their capacity is limited. They recognize words but not context, they’re blind to metadata, and in general they search and analyze documents at a superficial level. As a result, classic TAR models create large responsive sets with many documents that aren’t actually relevant, while leaving out many documents that are.
Advanced AI takes a much more comprehensive look, which makes it much more precise at capturing relevant documents and discarding irrelevant ones. It uses multiple layers of learning networks to incorporate a higher volume of data and broader range of data types and sources into its algorithms. It also utilizes natural language processing (NLP), which studies words in context to get a nuanced interpretation of meaning. This makes NLP much more accurate than older models when classifying responsiveness, privilege, and other categories.
Why It’s Valuable
Advanced AI enables legal teams to avoid the excessive delays and cost of reviewing thousands of irrelevant documents.
The benefits of a smaller and more precise responsive set are obvious: With fewer documents for eyes-on review, the whole effort is faster and less expensive.
And the need for these benefits becomes more pronounced every day. Datasets are swelling to millions of documents, while legal budgets are shrinking. Legal teams must reduce the responsive set in a cost-efficient and defensible way.
The cost of using advanced AI analytics is often outweighed by the savings it enables. TAR workflows that incorporate advanced AI have been approved by multiple courts and U.S. regulatory agencies for various matters, and a good eDiscovery partner will advise you on projected ROI and how the technology works so you can represent it with authority during litigation.
In the Real World
Advanced AI outperformed traditional TAR models during an HSR Second Request, using the same data and parameters.
During a recent Hart-Scott-Rodino Second Request, outside counsel for a Fortune 500 technology company used advanced AI to create a responsive set of documents for submission to the Department of Justice. The firm also ran two popular TAR models on the same data, using the same control set, to compare performance and inform the firm’s approach to future matters.
Advanced AI outperformed traditional TAR in several critical metrics:
- 430 fewer documents found to be potentially responsive than. the nearest alternative
- 50% fewer documents for privilege review, compared to classical TAR model
- 70% fewer foreign-language documents requiring translation and review, compared to one TAR model
- Overall savings of 18K review hours and $2M
AI for Single Matters: Refining Responsiveness
What It Is
Advanced AI builds responsive sets that are significantly smaller and more precise than those built by classic TAR models.
The most common technology-assisted review (TAR) models used in eDiscovery were designed in the 1970s, so their capacity is limited. They recognize words but not context, they’re blind to metadata, and in general they search and analyze documents at a superficial level. As a result, classic TAR models create large responsive sets with many documents that aren’t actually relevant, while leaving out many documents that are.
Advanced AI takes a much more comprehensive look, which makes it much more precise at capturing relevant documents and discarding irrelevant ones. It uses multiple layers of learning networks to incorporate a higher volume of data and broader range of data types and sources into its algorithms. It also utilizes natural language processing (NLP), which studies words in context to get a nuanced interpretation of meaning. This makes NLP much more accurate than older models when classifying responsiveness, privilege, and other categories.
Why It’s Valuable
Advanced AI enables legal teams to avoid the excessive delays and cost of reviewing thousands of irrelevant documents.
The benefits of a smaller and more precise responsive set are obvious: With fewer documents for eyes-on review, the whole effort is faster and less expensive.
And the need for these benefits becomes more pronounced every day. Datasets are swelling to millions of documents, while legal budgets are shrinking. Legal teams must reduce the responsive set in a cost-efficient and defensible way.
The cost of using advanced AI analytics is often outweighed by the savings it enables. TAR workflows that incorporate advanced AI have been approved by multiple courts and U.S. regulatory agencies for various matters, and a good eDiscovery partner will advise you on projected ROI and how the technology works so you can represent it with authority during litigation.
In the Real World
Advanced AI outperformed traditional TAR models during an HSR Second Request, using the same data and parameters.
During a recent Hart-Scott-Rodino Second Request, outside counsel for a Fortune 500 technology company used advanced AI to create a responsive set of documents for submission to the Department of Justice. The firm also ran two popular TAR models on the same data, using the same control set, to compare performance and inform the firm’s approach to future matters.
Advanced AI outperformed traditional TAR in several critical metrics:
- 430 fewer documents found to be potentially responsive than. the nearest alternative
- 50% fewer documents for privilege review, compared to classical TAR model
- 70% fewer foreign-language documents requiring translation and review, compared to one TAR model
- Overall savings of 18K review hours and $2M
AI for Single Matters: Refining Responsiveness
What It Is
Advanced AI builds responsive sets that are significantly smaller and more precise than those built by classic TAR models.
The most common technology-assisted review (TAR) models used in eDiscovery were designed in the 1970s, so their capacity is limited. They recognize words but not context, they’re blind to metadata, and in general they search and analyze documents at a superficial level. As a result, classic TAR models create large responsive sets with many documents that aren’t actually relevant, while leaving out many documents that are.
Advanced AI takes a much more comprehensive look, which makes it much more precise at capturing relevant documents and discarding irrelevant ones. It uses multiple layers of learning networks to incorporate a higher volume of data and broader range of data types and sources into its algorithms. It also utilizes natural language processing (NLP), which studies words in context to get a nuanced interpretation of meaning. This makes NLP much more accurate than older models when classifying responsiveness, privilege, and other categories.
Why It’s Valuable
Advanced AI enables legal teams to avoid the excessive delays and cost of reviewing thousands of irrelevant documents.
The benefits of a smaller and more precise responsive set are obvious: With fewer documents for eyes-on review, the whole effort is faster and less expensive.
And the need for these benefits becomes more pronounced every day. Datasets are swelling to millions of documents, while legal budgets are shrinking. Legal teams must reduce the responsive set in a cost-efficient and defensible way.
The cost of using advanced AI analytics is often outweighed by the savings it enables. TAR workflows that incorporate advanced AI have been approved by multiple courts and U.S. regulatory agencies for various matters, and a good eDiscovery partner will advise you on projected ROI and how the technology works so you can represent it with authority during litigation.
In the Real World
Advanced AI outperformed traditional TAR models during an HSR Second Request, using the same data and parameters.
During a recent Hart-Scott-Rodino Second Request, outside counsel for a Fortune 500 technology company used advanced AI to create a responsive set of documents for submission to the Department of Justice. The firm also ran two popular TAR models on the same data, using the same control set, to compare performance and inform the firm’s approach to future matters.
Advanced AI outperformed traditional TAR in several critical metrics:
- 430 fewer documents found to be potentially responsive than. the nearest alternative
- 50% fewer documents for privilege review, compared to classical TAR model
- 70% fewer foreign-language documents requiring translation and review, compared to one TAR model
- Overall savings of 18K review hours and $2M
AI for Multiple Matters: Reusing Work Product
What It Is
Work product reuse is the process of recalling and learning from old datasets to make document review more efficient and consistent from matter to matter.
In traditional eDiscovery, legal teams start from scratch on each new matter, regardless of how similar it is to the last one. Often this means reviewing and coding the same data again and again, as if for the first time. Not only is this redundant but, as data volumes grow, it’s becoming downright unfeasible.
Over the last decade, work product reuse emerged as a way to avoid the burden of repeated review. Until recently, the process was limited: Documents had to be identical between matters in order for teams to reuse coding.
Today, advancements in AI technology enable teams to reuse work product much more flexibly and effectively. Legal teams can unleash advanced AI to identify, reuse, and learn from the work product in millions of previously coded documents archived in old databases.
Why It’s Valuable
Even if documents are not identical among matters, past data and decisions are still a gold mine of knowledge.
This is especially true for classifications that generally stay the same from matter to matter, like junk, privilege, and sensitive information (e.g., privilege, personally identifiable information (PII), and trade secrets). Analytic tools that use advanced AI get smarter by analyzing previous attorney review decisions, metadata, language use, and other aspects and artifacts. This powers more precise assessments and recommendations when it encounters new documents.
When one of these documents comes up for review again, advanced AI uses that information to predict likelihood that it falls into one of the noted categories and can resurface its classification history along with it. Review attorneys are armed with historical and predictive data, making faster, data-driven decisions to apply document coding.
In the Real World
Using advanced AI analytics across matters enabled counsel at a global pharmaceutical company to save on document review and make strategic decisions sooner.
Inspired by the efficiency they achieved with advanced AI on a single matter, the company proceeded to use it on an additional 5 related matters. Each one proved to have thousands of documents in common with past or concurrent matters—more than 30,000 overlapping documents in some cases.
Past attorney work product was reused to reduce eyes-on review and improve consistency and accuracy:
Further insights followed, with immediate payoffs for review and case strategy. These included:
- 20K documents from one custodian were collected and processed across multiple matters, but only 10 documents ever actually made it to eyes-on review as potentially responsive documents.
- Another custodian’s documents were reviewed and produced across multiple matters and were classified as privilege 0% of the time.
AI for Multiple Matters: Reusing Work Product
What It Is
Work product reuse is the process of recalling and learning from old datasets to make document review more efficient and consistent from matter to matter.
In traditional eDiscovery, legal teams start from scratch on each new matter, regardless of how similar it is to the last one. Often this means reviewing and coding the same data again and again, as if for the first time. Not only is this redundant but, as data volumes grow, it’s becoming downright unfeasible.
Over the last decade, work product reuse emerged as a way to avoid the burden of repeated review. Until recently, the process was limited: Documents had to be identical between matters in order for teams to reuse coding.
Today, advancements in AI technology enable teams to reuse work product much more flexibly and effectively. Legal teams can unleash advanced AI to identify, reuse, and learn from the work product in millions of previously coded documents archived in old databases.
Why It’s Valuable
Even if documents are not identical among matters, past data and decisions are still a gold mine of knowledge.
This is especially true for classifications that generally stay the same from matter to matter, like junk, privilege, and sensitive information (e.g., privilege, personally identifiable information (PII), and trade secrets). Analytic tools that use advanced AI get smarter by analyzing previous attorney review decisions, metadata, language use, and other aspects and artifacts. This powers more precise assessments and recommendations when it encounters new documents.
When one of these documents comes up for review again, advanced AI uses that information to predict likelihood that it falls into one of the noted categories and can resurface its classification history along with it. Review attorneys are armed with historical and predictive data, making faster, data-driven decisions to apply document coding.
In the Real World
Using advanced AI analytics across matters enabled counsel at a global pharmaceutical company to save on document review and make strategic decisions sooner.
Inspired by the efficiency they achieved with advanced AI on a single matter, the company proceeded to use it on an additional 5 related matters. Each one proved to have thousands of documents in common with past or concurrent matters—more than 30,000 overlapping documents in some cases.
Past attorney work product was reused to reduce eyes-on review and improve consistency and accuracy:
Further insights followed, with immediate payoffs for review and case strategy. These included:
- 20K documents from one custodian were collected and processed across multiple matters, but only 10 documents ever actually made it to eyes-on review as potentially responsive documents.
- Another custodian’s documents were reviewed and produced across multiple matters and were classified as privilege 0% of the time.
AI for Multiple Matters: Reusing Work Product
What It Is
Work product reuse is the process of recalling and learning from old datasets to make document review more efficient and consistent from matter to matter.
In traditional eDiscovery, legal teams start from scratch on each new matter, regardless of how similar it is to the last one. Often this means reviewing and coding the same data again and again, as if for the first time. Not only is this redundant but, as data volumes grow, it’s becoming downright unfeasible.
Over the last decade, work product reuse emerged as a way to avoid the burden of repeated review. Until recently, the process was limited: Documents had to be identical between matters in order for teams to reuse coding.
Today, advancements in AI technology enable teams to reuse work product much more flexibly and effectively. Legal teams can unleash advanced AI to identify, reuse, and learn from the work product in millions of previously coded documents archived in old databases.
Why It’s Valuable
Even if documents are not identical among matters, past data and decisions are still a gold mine of knowledge.
This is especially true for classifications that generally stay the same from matter to matter, like junk, privilege, and sensitive information (e.g., privilege, personally identifiable information (PII), and trade secrets). Analytic tools that use advanced AI get smarter by analyzing previous attorney review decisions, metadata, language use, and other aspects and artifacts. This powers more precise assessments and recommendations when it encounters new documents.
When one of these documents comes up for review again, advanced AI uses that information to predict likelihood that it falls into one of the noted categories and can resurface its classification history along with it. Review attorneys are armed with historical and predictive data, making faster, data-driven decisions to apply document coding.
In the Real World
Using advanced AI analytics across matters enabled counsel at a global pharmaceutical company to save on document review and make strategic decisions sooner.
Inspired by the efficiency they achieved with advanced AI on a single matter, the company proceeded to use it on an additional 5 related matters. Each one proved to have thousands of documents in common with past or concurrent matters—more than 30,000 overlapping documents in some cases.
Past attorney work product was reused to reduce eyes-on review and improve consistency and accuracy:
Further insights followed, with immediate payoffs for review and case strategy. These included:
- 20K documents from one custodian were collected and processed across multiple matters, but only 10 documents ever actually made it to eyes-on review as potentially responsive documents.
- Another custodian’s documents were reviewed and produced across multiple matters and were classified as privilege 0% of the time.
AI for Multiple Matters: Reusing Work Product
What It Is
Work product reuse is the process of recalling and learning from old datasets to make document review more efficient and consistent from matter to matter.
In traditional eDiscovery, legal teams start from scratch on each new matter, regardless of how similar it is to the last one. Often this means reviewing and coding the same data again and again, as if for the first time. Not only is this redundant but, as data volumes grow, it’s becoming downright unfeasible.
Over the last decade, work product reuse emerged as a way to avoid the burden of repeated review. Until recently, the process was limited: Documents had to be identical between matters in order for teams to reuse coding.
Today, advancements in AI technology enable teams to reuse work product much more flexibly and effectively. Legal teams can unleash advanced AI to identify, reuse, and learn from the work product in millions of previously coded documents archived in old databases.
Why It’s Valuable
Even if documents are not identical among matters, past data and decisions are still a gold mine of knowledge.
This is especially true for classifications that generally stay the same from matter to matter, like junk, privilege, and sensitive information (e.g., privilege, personally identifiable information (PII), and trade secrets). Analytic tools that use advanced AI get smarter by analyzing previous attorney review decisions, metadata, language use, and other aspects and artifacts. This powers more precise assessments and recommendations when it encounters new documents.
When one of these documents comes up for review again, advanced AI uses that information to predict likelihood that it falls into one of the noted categories and can resurface its classification history along with it. Review attorneys are armed with historical and predictive data, making faster, data-driven decisions to apply document coding.
In the Real World
Using advanced AI analytics across matters enabled counsel at a global pharmaceutical company to save on document review and make strategic decisions sooner.
Inspired by the efficiency they achieved with advanced AI on a single matter, the company proceeded to use it on an additional 5 related matters. Each one proved to have thousands of documents in common with past or concurrent matters—more than 30,000 overlapping documents in some cases.
Past attorney work product was reused to reduce eyes-on review and improve consistency and accuracy:
Further insights followed, with immediate payoffs for review and case strategy. These included:
- 20K documents from one custodian were collected and processed across multiple matters, but only 10 documents ever actually made it to eyes-on review as potentially responsive documents.
- Another custodian’s documents were reviewed and produced across multiple matters and were classified as privilege 0% of the time.
AI for Multiple Matters: Reusing Work Product
What It Is
Work product reuse is the process of recalling and learning from old datasets to make document review more efficient and consistent from matter to matter.
In traditional eDiscovery, legal teams start from scratch on each new matter, regardless of how similar it is to the last one. Often this means reviewing and coding the same data again and again, as if for the first time. Not only is this redundant but, as data volumes grow, it’s becoming downright unfeasible.
Over the last decade, work product reuse emerged as a way to avoid the burden of repeated review. Until recently, the process was limited: Documents had to be identical between matters in order for teams to reuse coding.
Today, advancements in AI technology enable teams to reuse work product much more flexibly and effectively. Legal teams can unleash advanced AI to identify, reuse, and learn from the work product in millions of previously coded documents archived in old databases.
Why It’s Valuable
Even if documents are not identical among matters, past data and decisions are still a gold mine of knowledge.
This is especially true for classifications that generally stay the same from matter to matter, like junk, privilege, and sensitive information (e.g., privilege, personally identifiable information (PII), and trade secrets). Analytic tools that use advanced AI get smarter by analyzing previous attorney review decisions, metadata, language use, and other aspects and artifacts. This powers more precise assessments and recommendations when it encounters new documents.
When one of these documents comes up for review again, advanced AI uses that information to predict likelihood that it falls into one of the noted categories and can resurface its classification history along with it. Review attorneys are armed with historical and predictive data, making faster, data-driven decisions to apply document coding.
In the Real World
Using advanced AI analytics across matters enabled counsel at a global pharmaceutical company to save on document review and make strategic decisions sooner.
Inspired by the efficiency they achieved with advanced AI on a single matter, the company proceeded to use it on an additional 5 related matters. Each one proved to have thousands of documents in common with past or concurrent matters—more than 30,000 overlapping documents in some cases.
Past attorney work product was reused to reduce eyes-on review and improve consistency and accuracy:
Further insights followed, with immediate payoffs for review and case strategy. These included:
- 20K documents from one custodian were collected and processed across multiple matters, but only 10 documents ever actually made it to eyes-on review as potentially responsive documents.
- Another custodian’s documents were reviewed and produced across multiple matters and were classified as privilege 0% of the time.
AI for Multiple Matters: Reusing Work Product
What It Is
Work product reuse is the process of recalling and learning from old datasets to make document review more efficient and consistent from matter to matter.
In traditional eDiscovery, legal teams start from scratch on each new matter, regardless of how similar it is to the last one. Often this means reviewing and coding the same data again and again, as if for the first time. Not only is this redundant but, as data volumes grow, it’s becoming downright unfeasible.
Over the last decade, work product reuse emerged as a way to avoid the burden of repeated review. Until recently, the process was limited: Documents had to be identical between matters in order for teams to reuse coding.
Today, advancements in AI technology enable teams to reuse work product much more flexibly and effectively. Legal teams can unleash advanced AI to identify, reuse, and learn from the work product in millions of previously coded documents archived in old databases.
Why It’s Valuable
Even if documents are not identical among matters, past data and decisions are still a gold mine of knowledge.
This is especially true for classifications that generally stay the same from matter to matter, like junk, privilege, and sensitive information (e.g., privilege, personally identifiable information (PII), and trade secrets). Analytic tools that use advanced AI get smarter by analyzing previous attorney review decisions, metadata, language use, and other aspects and artifacts. This powers more precise assessments and recommendations when it encounters new documents.
When one of these documents comes up for review again, advanced AI uses that information to predict likelihood that it falls into one of the noted categories and can resurface its classification history along with it. Review attorneys are armed with historical and predictive data, making faster, data-driven decisions to apply document coding.
In the Real World
Using advanced AI analytics across matters enabled counsel at a global pharmaceutical company to save on document review and make strategic decisions sooner.
Inspired by the efficiency they achieved with advanced AI on a single matter, the company proceeded to use it on an additional 5 related matters. Each one proved to have thousands of documents in common with past or concurrent matters—more than 30,000 overlapping documents in some cases.
Past attorney work product was reused to reduce eyes-on review and improve consistency and accuracy:
Further insights followed, with immediate payoffs for review and case strategy. These included:
- 20K documents from one custodian were collected and processed across multiple matters, but only 10 documents ever actually made it to eyes-on review as potentially responsive documents.
- Another custodian’s documents were reviewed and produced across multiple matters and were classified as privilege 0% of the time.
AI for Multiple Matters: Reusing Work Product
What It Is
Work product reuse is the process of recalling and learning from old datasets to make document review more efficient and consistent from matter to matter.
In traditional eDiscovery, legal teams start from scratch on each new matter, regardless of how similar it is to the last one. Often this means reviewing and coding the same data again and again, as if for the first time. Not only is this redundant but, as data volumes grow, it’s becoming downright unfeasible.
Over the last decade, work product reuse emerged as a way to avoid the burden of repeated review. Until recently, the process was limited: Documents had to be identical between matters in order for teams to reuse coding.
Today, advancements in AI technology enable teams to reuse work product much more flexibly and effectively. Legal teams can unleash advanced AI to identify, reuse, and learn from the work product in millions of previously coded documents archived in old databases.
Why It’s Valuable
Even if documents are not identical among matters, past data and decisions are still a gold mine of knowledge.
This is especially true for classifications that generally stay the same from matter to matter, like junk, privilege, and sensitive information (e.g., privilege, personally identifiable information (PII), and trade secrets). Analytic tools that use advanced AI get smarter by analyzing previous attorney review decisions, metadata, language use, and other aspects and artifacts. This powers more precise assessments and recommendations when it encounters new documents.
When one of these documents comes up for review again, advanced AI uses that information to predict likelihood that it falls into one of the noted categories and can resurface its classification history along with it. Review attorneys are armed with historical and predictive data, making faster, data-driven decisions to apply document coding.
In the Real World
Using advanced AI analytics across matters enabled counsel at a global pharmaceutical company to save on document review and make strategic decisions sooner.
Inspired by the efficiency they achieved with advanced AI on a single matter, the company proceeded to use it on an additional 5 related matters. Each one proved to have thousands of documents in common with past or concurrent matters—more than 30,000 overlapping documents in some cases.
Past attorney work product was reused to reduce eyes-on review and improve consistency and accuracy:
Further insights followed, with immediate payoffs for review and case strategy. These included:
- 20K documents from one custodian were collected and processed across multiple matters, but only 10 documents ever actually made it to eyes-on review as potentially responsive documents.
- Another custodian’s documents were reviewed and produced across multiple matters and were classified as privilege 0% of the time.
AI for Multiple Matters: Reusing Work Product
What It Is
Work product reuse is the process of recalling and learning from old datasets to make document review more efficient and consistent from matter to matter.
In traditional eDiscovery, legal teams start from scratch on each new matter, regardless of how similar it is to the last one. Often this means reviewing and coding the same data again and again, as if for the first time. Not only is this redundant but, as data volumes grow, it’s becoming downright unfeasible.
Over the last decade, work product reuse emerged as a way to avoid the burden of repeated review. Until recently, the process was limited: Documents had to be identical between matters in order for teams to reuse coding.
Today, advancements in AI technology enable teams to reuse work product much more flexibly and effectively. Legal teams can unleash advanced AI to identify, reuse, and learn from the work product in millions of previously coded documents archived in old databases.
Why It’s Valuable
Even if documents are not identical among matters, past data and decisions are still a gold mine of knowledge.
This is especially true for classifications that generally stay the same from matter to matter, like junk, privilege, and sensitive information (e.g., privilege, personally identifiable information (PII), and trade secrets). Analytic tools that use advanced AI get smarter by analyzing previous attorney review decisions, metadata, language use, and other aspects and artifacts. This powers more precise assessments and recommendations when it encounters new documents.
When one of these documents comes up for review again, advanced AI uses that information to predict likelihood that it falls into one of the noted categories and can resurface its classification history along with it. Review attorneys are armed with historical and predictive data, making faster, data-driven decisions to apply document coding.
In the Real World
Using advanced AI analytics across matters enabled counsel at a global pharmaceutical company to save on document review and make strategic decisions sooner.
Inspired by the efficiency they achieved with advanced AI on a single matter, the company proceeded to use it on an additional 5 related matters. Each one proved to have thousands of documents in common with past or concurrent matters—more than 30,000 overlapping documents in some cases.
Past attorney work product was reused to reduce eyes-on review and improve consistency and accuracy:
Further insights followed, with immediate payoffs for review and case strategy. These included:
- 20K documents from one custodian were collected and processed across multiple matters, but only 10 documents ever actually made it to eyes-on review as potentially responsive documents.
- Another custodian’s documents were reviewed and produced across multiple matters and were classified as privilege 0% of the time.
AI for Multiple Matters: Reusing Work Product
What It Is
Work product reuse is the process of recalling and learning from old datasets to make document review more efficient and consistent from matter to matter.
In traditional eDiscovery, legal teams start from scratch on each new matter, regardless of how similar it is to the last one. Often this means reviewing and coding the same data again and again, as if for the first time. Not only is this redundant but, as data volumes grow, it’s becoming downright unfeasible.
Over the last decade, work product reuse emerged as a way to avoid the burden of repeated review. Until recently, the process was limited: Documents had to be identical between matters in order for teams to reuse coding.
Today, advancements in AI technology enable teams to reuse work product much more flexibly and effectively. Legal teams can unleash advanced AI to identify, reuse, and learn from the work product in millions of previously coded documents archived in old databases.
Why It’s Valuable
Even if documents are not identical among matters, past data and decisions are still a gold mine of knowledge.
This is especially true for classifications that generally stay the same from matter to matter, like junk, privilege, and sensitive information (e.g., privilege, personally identifiable information (PII), and trade secrets). Analytic tools that use advanced AI get smarter by analyzing previous attorney review decisions, metadata, language use, and other aspects and artifacts. This powers more precise assessments and recommendations when it encounters new documents.
When one of these documents comes up for review again, advanced AI uses that information to predict likelihood that it falls into one of the noted categories and can resurface its classification history along with it. Review attorneys are armed with historical and predictive data, making faster, data-driven decisions to apply document coding.
In the Real World
Using advanced AI analytics across matters enabled counsel at a global pharmaceutical company to save on document review and make strategic decisions sooner.
Inspired by the efficiency they achieved with advanced AI on a single matter, the company proceeded to use it on an additional 5 related matters. Each one proved to have thousands of documents in common with past or concurrent matters—more than 30,000 overlapping documents in some cases.
Past attorney work product was reused to reduce eyes-on review and improve consistency and accuracy:
Further insights followed, with immediate payoffs for review and case strategy. These included:
- 20K documents from one custodian were collected and processed across multiple matters, but only 10 documents ever actually made it to eyes-on review as potentially responsive documents.
- Another custodian’s documents were reviewed and produced across multiple matters and were classified as privilege 0% of the time.
AI for Multiple Matters: Reusing Work Product
What It Is
Work product reuse is the process of recalling and learning from old datasets to make document review more efficient and consistent from matter to matter.
In traditional eDiscovery, legal teams start from scratch on each new matter, regardless of how similar it is to the last one. Often this means reviewing and coding the same data again and again, as if for the first time. Not only is this redundant but, as data volumes grow, it’s becoming downright unfeasible.
Over the last decade, work product reuse emerged as a way to avoid the burden of repeated review. Until recently, the process was limited: Documents had to be identical between matters in order for teams to reuse coding.
Today, advancements in AI technology enable teams to reuse work product much more flexibly and effectively. Legal teams can unleash advanced AI to identify, reuse, and learn from the work product in millions of previously coded documents archived in old databases.
Why It’s Valuable
Even if documents are not identical among matters, past data and decisions are still a gold mine of knowledge.
This is especially true for classifications that generally stay the same from matter to matter, like junk, privilege, and sensitive information (e.g., privilege, personally identifiable information (PII), and trade secrets). Analytic tools that use advanced AI get smarter by analyzing previous attorney review decisions, metadata, language use, and other aspects and artifacts. This powers more precise assessments and recommendations when it encounters new documents.
When one of these documents comes up for review again, advanced AI uses that information to predict likelihood that it falls into one of the noted categories and can resurface its classification history along with it. Review attorneys are armed with historical and predictive data, making faster, data-driven decisions to apply document coding.
In the Real World
Using advanced AI analytics across matters enabled counsel at a global pharmaceutical company to save on document review and make strategic decisions sooner.
Inspired by the efficiency they achieved with advanced AI on a single matter, the company proceeded to use it on an additional 5 related matters. Each one proved to have thousands of documents in common with past or concurrent matters—more than 30,000 overlapping documents in some cases.
Past attorney work product was reused to reduce eyes-on review and improve consistency and accuracy:
Further insights followed, with immediate payoffs for review and case strategy. These included:
- 20K documents from one custodian were collected and processed across multiple matters, but only 10 documents ever actually made it to eyes-on review as potentially responsive documents.
- Another custodian’s documents were reviewed and produced across multiple matters and were classified as privilege 0% of the time.
AI for Multiple Matters: Reusing Work Product
What It Is
Work product reuse is the process of recalling and learning from old datasets to make document review more efficient and consistent from matter to matter.
In traditional eDiscovery, legal teams start from scratch on each new matter, regardless of how similar it is to the last one. Often this means reviewing and coding the same data again and again, as if for the first time. Not only is this redundant but, as data volumes grow, it’s becoming downright unfeasible.
Over the last decade, work product reuse emerged as a way to avoid the burden of repeated review. Until recently, the process was limited: Documents had to be identical between matters in order for teams to reuse coding.
Today, advancements in AI technology enable teams to reuse work product much more flexibly and effectively. Legal teams can unleash advanced AI to identify, reuse, and learn from the work product in millions of previously coded documents archived in old databases.
Why It’s Valuable
Even if documents are not identical among matters, past data and decisions are still a gold mine of knowledge.
This is especially true for classifications that generally stay the same from matter to matter, like junk, privilege, and sensitive information (e.g., privilege, personally identifiable information (PII), and trade secrets). Analytic tools that use advanced AI get smarter by analyzing previous attorney review decisions, metadata, language use, and other aspects and artifacts. This powers more precise assessments and recommendations when it encounters new documents.
When one of these documents comes up for review again, advanced AI uses that information to predict likelihood that it falls into one of the noted categories and can resurface its classification history along with it. Review attorneys are armed with historical and predictive data, making faster, data-driven decisions to apply document coding.
In the Real World
Using advanced AI analytics across matters enabled counsel at a global pharmaceutical company to save on document review and make strategic decisions sooner.
Inspired by the efficiency they achieved with advanced AI on a single matter, the company proceeded to use it on an additional 5 related matters. Each one proved to have thousands of documents in common with past or concurrent matters—more than 30,000 overlapping documents in some cases.
Past attorney work product was reused to reduce eyes-on review and improve consistency and accuracy:
Further insights followed, with immediate payoffs for review and case strategy. These included:
- 20K documents from one custodian were collected and processed across multiple matters, but only 10 documents ever actually made it to eyes-on review as potentially responsive documents.
- Another custodian’s documents were reviewed and produced across multiple matters and were classified as privilege 0% of the time.
AI for eDiscovery Programs: Portfolio-Level Analytics
What It Is
Portfolio-level analytics are metrics calculated by advanced AI based on an organization’s entire legal portfolio.
Like work product reuse, this involves leveraging and learning from previous work. But instead of looking at a select set of matters, advanced AI analytics are applied to an entire portfolio.
This “dashboard view” of all eDiscovery work product and data enables legal departments to make much more informed, strategic decisions—both on a specific matter and for the organization as a whole. It can help identify trends and make decisions that enhance efficiency and strategy while reducing legal costs and risks.
Why It’s Valuable
Legal teams can make numerous observations when they get a portfolio-level view of their work.
Not only does advanced AI make more accurate judgments the more data it ingests, it enables legal teams to act on trends they wouldn’t see otherwise. For example, they might find that particular custodians consistently have large volumes of attorney-client privilege or PII within their data. In the short term, this can help case teams plan for matter costs and make more informed eDiscovery burden arguments to courts and opposing counsel. In the long term, it can better inform their information governance and data retention policies.
A full portfolio view can also assist case teams even before collecting data for a current matter. They can look at what type of information resides in a custodial or data source collection and how it was previously coded, to help inform case strategy and control legal costs. Or an in-house legal team may discover patterns—such as increased litigation when specific custodians or data sources are involved in a matter—that can help organizations minimize risk and improve workplace compliance in the long term.
In the Real World
Access to a vast library of past matters enables advanced AI to make highly informed assessments of privilege.
A global technology company needed to conduct a privilege review on an expedited timeline. The traditional process of running privilege search terms identified more than 300,000 documents that would require review.
But the company had an ace up its sleeve: It had been using advanced AI analytics at the portfolio level for several months prior. With a comprehensive view of past matters, the AI model was able to make highly informed assessments of whether documents were likely to be privileged resulting in a 90% overall privilege review set reduction.
The approach also surfaced privileged documents that our old workflow may have missed, giving us greater confidence in the process. Advanced AI is now a standard component of all our Privilege reviews.
— eDiscovery Manager, Global Tech Company
AI for eDiscovery Programs: Portfolio-Level Analytics
What It Is
Portfolio-level analytics are metrics calculated by advanced AI based on an organization’s entire legal portfolio.
Like work product reuse, this involves leveraging and learning from previous work. But instead of looking at a select set of matters, advanced AI analytics are applied to an entire portfolio.
This “dashboard view” of all eDiscovery work product and data enables legal departments to make much more informed, strategic decisions—both on a specific matter and for the organization as a whole. It can help identify trends and make decisions that enhance efficiency and strategy while reducing legal costs and risks.
Why It’s Valuable
Legal teams can make numerous observations when they get a portfolio-level view of their work.
Not only does advanced AI make more accurate judgments the more data it ingests, it enables legal teams to act on trends they wouldn’t see otherwise. For example, they might find that particular custodians consistently have large volumes of attorney-client privilege or PII within their data. In the short term, this can help case teams plan for matter costs and make more informed eDiscovery burden arguments to courts and opposing counsel. In the long term, it can better inform their information governance and data retention policies.
A full portfolio view can also assist case teams even before collecting data for a current matter. They can look at what type of information resides in a custodial or data source collection and how it was previously coded, to help inform case strategy and control legal costs. Or an in-house legal team may discover patterns—such as increased litigation when specific custodians or data sources are involved in a matter—that can help organizations minimize risk and improve workplace compliance in the long term.
In the Real World
Access to a vast library of past matters enables advanced AI to make highly informed assessments of privilege.
A global technology company needed to conduct a privilege review on an expedited timeline. The traditional process of running privilege search terms identified more than 300,000 documents that would require review.
But the company had an ace up its sleeve: It had been using advanced AI analytics at the portfolio level for several months prior. With a comprehensive view of past matters, the AI model was able to make highly informed assessments of whether documents were likely to be privileged resulting in a 90% overall privilege review set reduction.
The approach also surfaced privileged documents that our old workflow may have missed, giving us greater confidence in the process. Advanced AI is now a standard component of all our Privilege reviews.
— eDiscovery Manager, Global Tech Company
AI for eDiscovery Programs: Portfolio-Level Analytics
What It Is
Portfolio-level analytics are metrics calculated by advanced AI based on an organization’s entire legal portfolio.
Like work product reuse, this involves leveraging and learning from previous work. But instead of looking at a select set of matters, advanced AI analytics are applied to an entire portfolio.
This “dashboard view” of all eDiscovery work product and data enables legal departments to make much more informed, strategic decisions—both on a specific matter and for the organization as a whole. It can help identify trends and make decisions that enhance efficiency and strategy while reducing legal costs and risks.
Why It’s Valuable
Legal teams can make numerous observations when they get a portfolio-level view of their work.
Not only does advanced AI make more accurate judgments the more data it ingests, it enables legal teams to act on trends they wouldn’t see otherwise. For example, they might find that particular custodians consistently have large volumes of attorney-client privilege or PII within their data. In the short term, this can help case teams plan for matter costs and make more informed eDiscovery burden arguments to courts and opposing counsel. In the long term, it can better inform their information governance and data retention policies.
A full portfolio view can also assist case teams even before collecting data for a current matter. They can look at what type of information resides in a custodial or data source collection and how it was previously coded, to help inform case strategy and control legal costs. Or an in-house legal team may discover patterns—such as increased litigation when specific custodians or data sources are involved in a matter—that can help organizations minimize risk and improve workplace compliance in the long term.
In the Real World
Access to a vast library of past matters enables advanced AI to make highly informed assessments of privilege.
A global technology company needed to conduct a privilege review on an expedited timeline. The traditional process of running privilege search terms identified more than 300,000 documents that would require review.
But the company had an ace up its sleeve: It had been using advanced AI analytics at the portfolio level for several months prior. With a comprehensive view of past matters, the AI model was able to make highly informed assessments of whether documents were likely to be privileged resulting in a 90% overall privilege review set reduction.
The approach also surfaced privileged documents that our old workflow may have missed, giving us greater confidence in the process. Advanced AI is now a standard component of all our Privilege reviews.
— eDiscovery Manager, Global Tech Company
AI for eDiscovery Programs: Portfolio-Level Analytics
What It Is
Portfolio-level analytics are metrics calculated by advanced AI based on an organization’s entire legal portfolio.
Like work product reuse, this involves leveraging and learning from previous work. But instead of looking at a select set of matters, advanced AI analytics are applied to an entire portfolio.
This “dashboard view” of all eDiscovery work product and data enables legal departments to make much more informed, strategic decisions—both on a specific matter and for the organization as a whole. It can help identify trends and make decisions that enhance efficiency and strategy while reducing legal costs and risks.
Why It’s Valuable
Legal teams can make numerous observations when they get a portfolio-level view of their work.
Not only does advanced AI make more accurate judgments the more data it ingests, it enables legal teams to act on trends they wouldn’t see otherwise. For example, they might find that particular custodians consistently have large volumes of attorney-client privilege or PII within their data. In the short term, this can help case teams plan for matter costs and make more informed eDiscovery burden arguments to courts and opposing counsel. In the long term, it can better inform their information governance and data retention policies.
A full portfolio view can also assist case teams even before collecting data for a current matter. They can look at what type of information resides in a custodial or data source collection and how it was previously coded, to help inform case strategy and control legal costs. Or an in-house legal team may discover patterns—such as increased litigation when specific custodians or data sources are involved in a matter—that can help organizations minimize risk and improve workplace compliance in the long term.
In the Real World
Access to a vast library of past matters enables advanced AI to make highly informed assessments of privilege.
A global technology company needed to conduct a privilege review on an expedited timeline. The traditional process of running privilege search terms identified more than 300,000 documents that would require review.
But the company had an ace up its sleeve: It had been using advanced AI analytics at the portfolio level for several months prior. With a comprehensive view of past matters, the AI model was able to make highly informed assessments of whether documents were likely to be privileged resulting in a 90% overall privilege review set reduction.
The approach also surfaced privileged documents that our old workflow may have missed, giving us greater confidence in the process. Advanced AI is now a standard component of all our Privilege reviews.
— eDiscovery Manager, Global Tech Company
AI for eDiscovery Programs: Portfolio-Level Analytics
What It Is
Portfolio-level analytics are metrics calculated by advanced AI based on an organization’s entire legal portfolio.
Like work product reuse, this involves leveraging and learning from previous work. But instead of looking at a select set of matters, advanced AI analytics are applied to an entire portfolio.
This “dashboard view” of all eDiscovery work product and data enables legal departments to make much more informed, strategic decisions—both on a specific matter and for the organization as a whole. It can help identify trends and make decisions that enhance efficiency and strategy while reducing legal costs and risks.
Why It’s Valuable
Legal teams can make numerous observations when they get a portfolio-level view of their work.
Not only does advanced AI make more accurate judgments the more data it ingests, it enables legal teams to act on trends they wouldn’t see otherwise. For example, they might find that particular custodians consistently have large volumes of attorney-client privilege or PII within their data. In the short term, this can help case teams plan for matter costs and make more informed eDiscovery burden arguments to courts and opposing counsel. In the long term, it can better inform their information governance and data retention policies.
A full portfolio view can also assist case teams even before collecting data for a current matter. They can look at what type of information resides in a custodial or data source collection and how it was previously coded, to help inform case strategy and control legal costs. Or an in-house legal team may discover patterns—such as increased litigation when specific custodians or data sources are involved in a matter—that can help organizations minimize risk and improve workplace compliance in the long term.
In the Real World
Access to a vast library of past matters enables advanced AI to make highly informed assessments of privilege.
A global technology company needed to conduct a privilege review on an expedited timeline. The traditional process of running privilege search terms identified more than 300,000 documents that would require review.
But the company had an ace up its sleeve: It had been using advanced AI analytics at the portfolio level for several months prior. With a comprehensive view of past matters, the AI model was able to make highly informed assessments of whether documents were likely to be privileged resulting in a 90% overall privilege review set reduction.
The approach also surfaced privileged documents that our old workflow may have missed, giving us greater confidence in the process. Advanced AI is now a standard component of all our Privilege reviews.
— eDiscovery Manager, Global Tech Company
AI for eDiscovery Programs: Portfolio-Level Analytics
What It Is
Portfolio-level analytics are metrics calculated by advanced AI based on an organization’s entire legal portfolio.
Like work product reuse, this involves leveraging and learning from previous work. But instead of looking at a select set of matters, advanced AI analytics are applied to an entire portfolio.
This “dashboard view” of all eDiscovery work product and data enables legal departments to make much more informed, strategic decisions—both on a specific matter and for the organization as a whole. It can help identify trends and make decisions that enhance efficiency and strategy while reducing legal costs and risks.
Why It’s Valuable
Legal teams can make numerous observations when they get a portfolio-level view of their work.
Not only does advanced AI make more accurate judgments the more data it ingests, it enables legal teams to act on trends they wouldn’t see otherwise. For example, they might find that particular custodians consistently have large volumes of attorney-client privilege or PII within their data. In the short term, this can help case teams plan for matter costs and make more informed eDiscovery burden arguments to courts and opposing counsel. In the long term, it can better inform their information governance and data retention policies.
A full portfolio view can also assist case teams even before collecting data for a current matter. They can look at what type of information resides in a custodial or data source collection and how it was previously coded, to help inform case strategy and control legal costs. Or an in-house legal team may discover patterns—such as increased litigation when specific custodians or data sources are involved in a matter—that can help organizations minimize risk and improve workplace compliance in the long term.
In the Real World
Access to a vast library of past matters enables advanced AI to make highly informed assessments of privilege.
A global technology company needed to conduct a privilege review on an expedited timeline. The traditional process of running privilege search terms identified more than 300,000 documents that would require review.
But the company had an ace up its sleeve: It had been using advanced AI analytics at the portfolio level for several months prior. With a comprehensive view of past matters, the AI model was able to make highly informed assessments of whether documents were likely to be privileged resulting in a 90% overall privilege review set reduction.
The approach also surfaced privileged documents that our old workflow may have missed, giving us greater confidence in the process. Advanced AI is now a standard component of all our Privilege reviews.
— eDiscovery Manager, Global Tech Company
AI for eDiscovery Programs: Portfolio-Level Analytics
What It Is
Portfolio-level analytics are metrics calculated by advanced AI based on an organization’s entire legal portfolio.
Like work product reuse, this involves leveraging and learning from previous work. But instead of looking at a select set of matters, advanced AI analytics are applied to an entire portfolio.
This “dashboard view” of all eDiscovery work product and data enables legal departments to make much more informed, strategic decisions—both on a specific matter and for the organization as a whole. It can help identify trends and make decisions that enhance efficiency and strategy while reducing legal costs and risks.
Why It’s Valuable
Legal teams can make numerous observations when they get a portfolio-level view of their work.
Not only does advanced AI make more accurate judgments the more data it ingests, it enables legal teams to act on trends they wouldn’t see otherwise. For example, they might find that particular custodians consistently have large volumes of attorney-client privilege or PII within their data. In the short term, this can help case teams plan for matter costs and make more informed eDiscovery burden arguments to courts and opposing counsel. In the long term, it can better inform their information governance and data retention policies.
A full portfolio view can also assist case teams even before collecting data for a current matter. They can look at what type of information resides in a custodial or data source collection and how it was previously coded, to help inform case strategy and control legal costs. Or an in-house legal team may discover patterns—such as increased litigation when specific custodians or data sources are involved in a matter—that can help organizations minimize risk and improve workplace compliance in the long term.
In the Real World
Access to a vast library of past matters enables advanced AI to make highly informed assessments of privilege.
A global technology company needed to conduct a privilege review on an expedited timeline. The traditional process of running privilege search terms identified more than 300,000 documents that would require review.
But the company had an ace up its sleeve: It had been using advanced AI analytics at the portfolio level for several months prior. With a comprehensive view of past matters, the AI model was able to make highly informed assessments of whether documents were likely to be privileged resulting in a 90% overall privilege review set reduction.
The approach also surfaced privileged documents that our old workflow may have missed, giving us greater confidence in the process. Advanced AI is now a standard component of all our Privilege reviews.
— eDiscovery Manager, Global Tech Company
AI for eDiscovery Programs: Portfolio-Level Analytics
What It Is
Portfolio-level analytics are metrics calculated by advanced AI based on an organization’s entire legal portfolio.
Like work product reuse, this involves leveraging and learning from previous work. But instead of looking at a select set of matters, advanced AI analytics are applied to an entire portfolio.
This “dashboard view” of all eDiscovery work product and data enables legal departments to make much more informed, strategic decisions—both on a specific matter and for the organization as a whole. It can help identify trends and make decisions that enhance efficiency and strategy while reducing legal costs and risks.
Why It’s Valuable
Legal teams can make numerous observations when they get a portfolio-level view of their work.
Not only does advanced AI make more accurate judgments the more data it ingests, it enables legal teams to act on trends they wouldn’t see otherwise. For example, they might find that particular custodians consistently have large volumes of attorney-client privilege or PII within their data. In the short term, this can help case teams plan for matter costs and make more informed eDiscovery burden arguments to courts and opposing counsel. In the long term, it can better inform their information governance and data retention policies.
A full portfolio view can also assist case teams even before collecting data for a current matter. They can look at what type of information resides in a custodial or data source collection and how it was previously coded, to help inform case strategy and control legal costs. Or an in-house legal team may discover patterns—such as increased litigation when specific custodians or data sources are involved in a matter—that can help organizations minimize risk and improve workplace compliance in the long term.
In the Real World
Access to a vast library of past matters enables advanced AI to make highly informed assessments of privilege.
A global technology company needed to conduct a privilege review on an expedited timeline. The traditional process of running privilege search terms identified more than 300,000 documents that would require review.
But the company had an ace up its sleeve: It had been using advanced AI analytics at the portfolio level for several months prior. With a comprehensive view of past matters, the AI model was able to make highly informed assessments of whether documents were likely to be privileged resulting in a 90% overall privilege review set reduction.
The approach also surfaced privileged documents that our old workflow may have missed, giving us greater confidence in the process. Advanced AI is now a standard component of all our Privilege reviews.
— eDiscovery Manager, Global Tech Company
AI for eDiscovery Programs: Portfolio-Level Analytics
What It Is
Portfolio-level analytics are metrics calculated by advanced AI based on an organization’s entire legal portfolio.
Like work product reuse, this involves leveraging and learning from previous work. But instead of looking at a select set of matters, advanced AI analytics are applied to an entire portfolio.
This “dashboard view” of all eDiscovery work product and data enables legal departments to make much more informed, strategic decisions—both on a specific matter and for the organization as a whole. It can help identify trends and make decisions that enhance efficiency and strategy while reducing legal costs and risks.
Why It’s Valuable
Legal teams can make numerous observations when they get a portfolio-level view of their work.
Not only does advanced AI make more accurate judgments the more data it ingests, it enables legal teams to act on trends they wouldn’t see otherwise. For example, they might find that particular custodians consistently have large volumes of attorney-client privilege or PII within their data. In the short term, this can help case teams plan for matter costs and make more informed eDiscovery burden arguments to courts and opposing counsel. In the long term, it can better inform their information governance and data retention policies.
A full portfolio view can also assist case teams even before collecting data for a current matter. They can look at what type of information resides in a custodial or data source collection and how it was previously coded, to help inform case strategy and control legal costs. Or an in-house legal team may discover patterns—such as increased litigation when specific custodians or data sources are involved in a matter—that can help organizations minimize risk and improve workplace compliance in the long term.
In the Real World
Access to a vast library of past matters enables advanced AI to make highly informed assessments of privilege.
A global technology company needed to conduct a privilege review on an expedited timeline. The traditional process of running privilege search terms identified more than 300,000 documents that would require review.
But the company had an ace up its sleeve: It had been using advanced AI analytics at the portfolio level for several months prior. With a comprehensive view of past matters, the AI model was able to make highly informed assessments of whether documents were likely to be privileged resulting in a 90% overall privilege review set reduction.
The approach also surfaced privileged documents that our old workflow may have missed, giving us greater confidence in the process. Advanced AI is now a standard component of all our Privilege reviews.
— eDiscovery Manager, Global Tech Company
AI for eDiscovery Programs: Portfolio-Level Analytics
What It Is
Portfolio-level analytics are metrics calculated by advanced AI based on an organization’s entire legal portfolio.
Like work product reuse, this involves leveraging and learning from previous work. But instead of looking at a select set of matters, advanced AI analytics are applied to an entire portfolio.
This “dashboard view” of all eDiscovery work product and data enables legal departments to make much more informed, strategic decisions—both on a specific matter and for the organization as a whole. It can help identify trends and make decisions that enhance efficiency and strategy while reducing legal costs and risks.
Why It’s Valuable
Legal teams can make numerous observations when they get a portfolio-level view of their work.
Not only does advanced AI make more accurate judgments the more data it ingests, it enables legal teams to act on trends they wouldn’t see otherwise. For example, they might find that particular custodians consistently have large volumes of attorney-client privilege or PII within their data. In the short term, this can help case teams plan for matter costs and make more informed eDiscovery burden arguments to courts and opposing counsel. In the long term, it can better inform their information governance and data retention policies.
A full portfolio view can also assist case teams even before collecting data for a current matter. They can look at what type of information resides in a custodial or data source collection and how it was previously coded, to help inform case strategy and control legal costs. Or an in-house legal team may discover patterns—such as increased litigation when specific custodians or data sources are involved in a matter—that can help organizations minimize risk and improve workplace compliance in the long term.
In the Real World
Access to a vast library of past matters enables advanced AI to make highly informed assessments of privilege.
A global technology company needed to conduct a privilege review on an expedited timeline. The traditional process of running privilege search terms identified more than 300,000 documents that would require review.
But the company had an ace up its sleeve: It had been using advanced AI analytics at the portfolio level for several months prior. With a comprehensive view of past matters, the AI model was able to make highly informed assessments of whether documents were likely to be privileged resulting in a 90% overall privilege review set reduction.
The approach also surfaced privileged documents that our old workflow may have missed, giving us greater confidence in the process. Advanced AI is now a standard component of all our Privilege reviews.
— eDiscovery Manager, Global Tech Company
AI for eDiscovery Programs: Portfolio-Level Analytics
What It Is
Portfolio-level analytics are metrics calculated by advanced AI based on an organization’s entire legal portfolio.
Like work product reuse, this involves leveraging and learning from previous work. But instead of looking at a select set of matters, advanced AI analytics are applied to an entire portfolio.
This “dashboard view” of all eDiscovery work product and data enables legal departments to make much more informed, strategic decisions—both on a specific matter and for the organization as a whole. It can help identify trends and make decisions that enhance efficiency and strategy while reducing legal costs and risks.
Why It’s Valuable
Legal teams can make numerous observations when they get a portfolio-level view of their work.
Not only does advanced AI make more accurate judgments the more data it ingests, it enables legal teams to act on trends they wouldn’t see otherwise. For example, they might find that particular custodians consistently have large volumes of attorney-client privilege or PII within their data. In the short term, this can help case teams plan for matter costs and make more informed eDiscovery burden arguments to courts and opposing counsel. In the long term, it can better inform their information governance and data retention policies.
A full portfolio view can also assist case teams even before collecting data for a current matter. They can look at what type of information resides in a custodial or data source collection and how it was previously coded, to help inform case strategy and control legal costs. Or an in-house legal team may discover patterns—such as increased litigation when specific custodians or data sources are involved in a matter—that can help organizations minimize risk and improve workplace compliance in the long term.
In the Real World
Access to a vast library of past matters enables advanced AI to make highly informed assessments of privilege.
A global technology company needed to conduct a privilege review on an expedited timeline. The traditional process of running privilege search terms identified more than 300,000 documents that would require review.
But the company had an ace up its sleeve: It had been using advanced AI analytics at the portfolio level for several months prior. With a comprehensive view of past matters, the AI model was able to make highly informed assessments of whether documents were likely to be privileged resulting in a 90% overall privilege review set reduction.
The approach also surfaced privileged documents that our old workflow may have missed, giving us greater confidence in the process. Advanced AI is now a standard component of all our Privilege reviews.
— eDiscovery Manager, Global Tech Company
Want to Know More?
Looking at single-matter, multiple-matter, and portfolio-level applications of advanced AI is a great way to understand this new technology and its potential to enhance document review. But advanced AI can support eDiscovery in many other ways. And exploring its potential doesn’t have to be an all-or-nothing proposition. Curious teams and attorneys can start small and build up familiarity and utilization incrementally.
We hope this answers at least some of your questions about how advancements in AI affect the legal industry and your work and practice in particular. For further answers, insights, and examples, visit our Review Intelligence page or fill out the form to get in touch.