New Rules, New Tools: AI and Compliance
June 28, 2021
|
By:
We live in the era of Big Data. The exponential pace of technological development continues to generate immense amounts of digital information that can be analyzed, sorted, and utilized in previously impossible ways. In this world of artificial intelligence (AI), machine learning, and other advanced technologies, questions of privacy, government regulations, and compliance have taken on a new prominence across industries of all kinds.
With this in mind, H5 recently convened a panel of experts to discuss the latest compliance challenges that organizations are facing today, as well as ways that AI can be used to address those challenges. Some key topics covered in the discussion included:
- Understanding use cases involving technical approaches to data classification.
- Exploring emerging data classification methods and approach.
- Setting expectations within your organization for the deployment of AI technology.
- Keeping an AI solution compliant.
- Preventing introducing bias into your AI models.
The panel included Timia Moore, strategic risk assessment manager for Wells Fargo; Kimberly Pack, associate general counsel of compliance for Anheuser-Busch; Alex Lakatos, partner at Mayer Brown; and Eric Pender, engagement manager at H5; The conversation was moderated by Doug Austin, editor of the eDiscovery Today blog.
Compliance Challenges Organizations Are Facing Today
The rapidly evolving regulatory landscape, vastly increased data volumes and sources, and stringent new privacy laws present unique new challenges to today’s businesses. Whereas in the recent past it may have seemed liked regulatory bodies were often in a defensive position, forced to play catch-up as powerful new technologies took the field, these agencies are increasingly using their own tech to go on the offensive.
This is particularly true in the banking industry and broader financial sector. “With the advent of fintech and technology like AI, regulators are moving from this reactive mode into a more proactive mode,” said Timia Moore, strategic risk assessment manager for Wells Fargo. But the trend is not limited to banking and finance. “It’s not industry specific,” she said. “I think regulators are really looking to be more proactive and figure out how to identify and assess issues, because ultimately they’re concerned about the consumer, which all of our companies are and should be as well.”
Indeed, growing demand by consumers for increased privacy and better protection of their personal data is a key driver of new regulations around the world, including the General Data Protection Regulation (GDPR) in the European Union and the California Consumer Privacy Act (CCPA) and various similar laws in the United States. It’s also one of the biggest compliance challenges facing organizations today, as cyber attacks are now faster, more aggressive, and more sophisticated than ever before.
Other challenges highlighted by the panel included:
- Siloed departments that limit communications and visibility within organizations
- A dearth of subject matter expertise
- The possibility of simultaneous AI requests from multiple regulatory agencies
- A more remote and dispersed workforce due to the pandemic
Use Cases for AI and Compliance
In order to meet these challenges head on, companies are increasingly turning to AI to help them comply with new regulations. Some companies are partnering with technology specialists to meet their AI needs, while some are building their own systems.
Anheuser-Busch is one such company that is using an AI system to meet compliance standards. As Kimberly Pack, associate general counsel of compliance for Anheuser-Busch, described it: “One of the things that we’re super proud of is our proprietary AI data analyst system BrewRight. We use that data for Foreign Corrupt Practices Act compliance. We use it for investigations management. We use it for alcohol beverage law compliance.”
She also pointed out that the BrewRight AI system is useful for discovering internal malfeasance as well. “Just general employee credit card abuse…We can even identify those kinds of things,” Pack said. “We’re actively looking for outlier behavior, strange patterns or new activity. As companies, we have this data, and so the question is how are we using it, and artificial intelligence is a great way for us to start being able to identify and mitigate some risks that we have.”
Artificial intelligence can also play a key role in reducing the burden from alerts related to potential compliance issues or other kinds of wrongdoing. The trick, according to Alex Lakatos, partner at Mayer Brown, is tuning the system to the right level of sensitivity—and then letting it learn from there. “If you set it to be too sensitive, you’re going to be drowned in alerts and you can’t make sense of them,” Lakatos said. “You set it too far in the other direction, you only get the instances of the really, really bad conduct. But AI, because it is a learning tool, can become smarter about which alerts get triggered.”
Lakatos also pointed out that when it comes to the kind of explanations for illegal behaviors that regulators usually want to see, AI is not capable of providing those answers. “AI doesn’t work on a theory,” he said. “AI just works on correlation.” That’s where having some smart people working in tandem with your AI comes in handy. “Regulators get more comfortable with a little bit of theory behind it.”
H5 has identified at least a dozen areas related to compliance where AI can be of assistance, including: key document retention and categorization, personal identifiable information (PII) location and remediation, first-line level reviews of alerts, and policy applicability and risk identification.
Data Classification, Methods, and Approaches
There are various methods and approaches to data classification, including machine learning, linguistic modeling, sentiment analysis, name normalization, and personal data detection. Choosing the right one depends on what companies want their AI to do.
“That’s why it’s really important to have a holistic program management style approach to this,” said Eric Pender, engagement manager at H5. “Because there are so many different ways that you can approach a lot of these problems.”
Supervised machine learning models, for instance, ingest data that’s already been categorized, which makes them great at making predictions and predictive models. Unsupervised machine learning models, on the other hand, which take in unlabeled, uncategorized information, are really good at data pattern and structure recognition.
“Ultimately, I think this comes down to the question of what action you want to take on your data,” Pender said. “And what version of modeling is going to be best suited to getting you there.”
Setting Expectations for AI Deployment
Once you’ve determined the type of data classification that best suits your needs, it’s crucial to set expectations for the AI deployment within your company. This process includes third-party evaluation, procurement, testing, and data processing agreements. Buying an off-the shelf solution is a possibility, though some organizations—especially large ones—may have the resources to build their own. It’s also possible to create a solution that features elements of both. In either case, obtaining C-suite buy-in is a critical step that should not be overlooked. And to maintain trust, it’s important to properly notify workers throughout the organization and remain transparent throughout the process.
Allowing enough time for proper proof of concept evaluation is also key. When it comes to creating a timeline for deploying AI within an organization, “it’s really important for folks to be patient,” according to Pender. “People who are new to AI sometimes have this perception that they’re going to buy AI and they’re going to plug it in and it just works. But you really have to take time to train the models, especially if you’re talking about structured algorithms and you need to input classified data.”
Education, documentation, and training are also key aspects of setting expectations for AI deployment. Bear in mind, at its heart implementing an AI system is a form of change management.
“Think about your organization and the culture, and how well your employees or impacted team members receive change,” said Timia Moore of Wells Fargo. “Sometimes—if you are developing that change internally, if they’re at the table, if they have a voice, if they feel they’re a meaningful part of it—it’s a lot easier than if you just have some cowboy vendor come in and say, ‘We have the answer to your problems. Here it is, just do what we say.’”
Keeping AI Solutions Compliant and Avoiding Bias
When deploying an AI system, the last area of consideration discussed by the panel was how to keep the AI solution itself compliant and free of bias. Best practices include ongoing monitoring of the system, A/B testing, and mitigating attacks on the AI model.
It’s also important to always keep in mind that AI systems are inherently dependent on their own training data. In other words, these systems are only as good as their inputs, and it’s crucial to make sure biases aren’t baked into the AI from the beginning. And once the system is up and running—and learning—it’s important to check in on it regularly.
“There’s an old computer saying, ‘Garbage in, garbage out,’ said Lakatos. “The thing with AI is people have so much faith in it that it is become more of ‘garbage in, gospel out.’ If the AI says it, it must be true…and that’s something to be cautious of.”
In today’s digital world, AI systems are becoming more and more integral to compliance and a host of other business functions. Educating yourself and making sure your company has a plan for the future are essential steps to take right away.
The entire H5 webcast, “New Rules, New Tools: AI and Compliance,” can be viewed here.
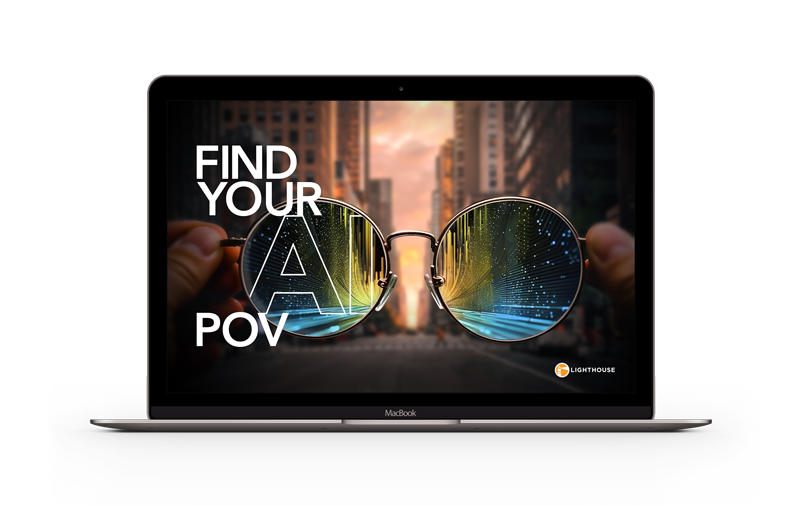