Big Data Challenges in eDiscovery (and How AI-Based Analytics Can Help)
June 7, 2021
By:
It’s no secret that big data can mean big challenges in the eDiscovery world. Data volumes and sources are exploding year after year, in part due to a global shift to digital forms of communication in working environments (think emails, chat messages, and cloud-based collaboration tools vs. phone calls, in-person meetings, and paper memorandums, etc.) as well as the rise of the Cloud (which provides cheaper, more flexible, and virtually limitless data storage capabilities).
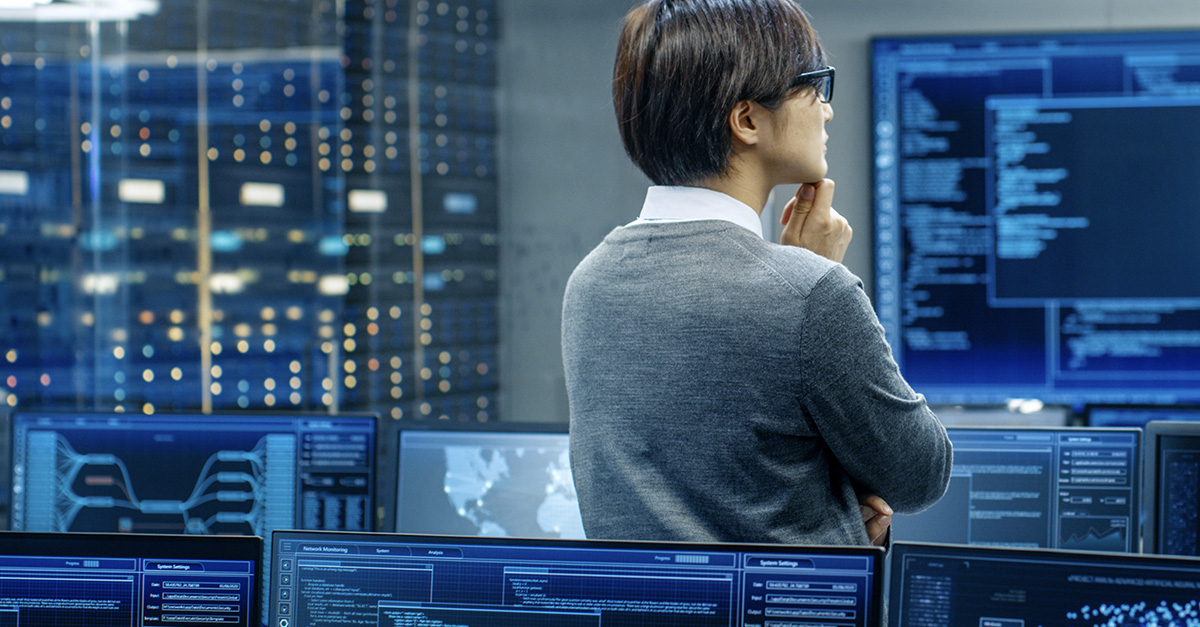
This means that with every new litigation or investigation requiring discovery, counsel must collect massive amounts of potentially relevant digital evidence, host it, process it, identify the relevant information within it (as well as pinpoint any sensitive or protected information within that relevant data) and then produce that relevant data to the opposing side. Traditionally, this process then starts all over again with the next litigation – often beginning back at square one in a vacuum by collecting the exact same data for the new matter, without any of the insights or attorney work product gained from the previous matter.
This endless cycle is not sustainable as data volumes continue to grow exponentially. Fortunately, just as advances in technology have led to increasing data volumes, advances in artificial intelligence (AI) technology can help tackle big data challenges. Newer analytics technology can now use multiple algorithms to analyze millions of data points across an organization’s entire legal portfolio (including metadata, text, past attorney work product, etc.) and provide counsel with insights that can improve efficiency and curb the endless cycle of re-inventing the wheel on each new matter.
In this post, I’ll outline the four main challenges big data can pose in an eDiscovery environment (also called “The Four Vs”) and explain how cutting-edge big data analytics tools can help tackle them.
The “Four Vs” of Big Data Challenges in eDiscovery
1. The volume, or scale of data
As noted above, a primary challenge in matters involving discovery is the sheer amount of data generated by employees and organizations as a whole. For reference, most companies in the U.S. currently have at least 100 terabytes of data stored, and it is estimated that by 2025, worldwide data will grow 61 percent to 175 zettabytes.
As organizations and individuals create more data, data volumes for even routine or small eDiscovery matters are exploding in correlation. Unfortunately, court discovery deadlines and opposing counsel production expectations rarely adjust to accommodate this ever-growing surge in data. This can put organizations and outside counsel in an impossible position if they don’t have a defensible and efficient method to cull irrelevant data and/or accurately identify important categories of data within large, complex data sets. Being forced to manually review vast amounts of information within an unrealistic time period can quickly become a pressure cooker for critical mistakes – where review teams miss important information within a dataset and thereby either produce damaging or sensitive information to the opposing side (e.g., attorney-client privilege, protected health information, trade secrets, non-relevant information, etc.) or in the inverse, fail to find and produce requested relevant information.
To overcome this challenge, counsel (both in-house and outside counsel) need better ways to retain and analyze data – which is exactly where newer AI-enabled analytics technology (which can better manage large volumes of data) can help. The AI-based analytics technology being built right now is developed for scale, meaning new technology can handle large caseloads, easily add data, and create feedback loops that run in real time. Each document that is reviewed feeds into the algorithm to make the analysis even more precise moving forward. This differs from older analytics platforms, which were not engineered to meet the challenges of data volumes today – resulting in review delays or worse, inaccurate output that leads to critical mistakes.
2. The variety, or different forms of data
In addition to the volume of data increasing today, the diversity of data sources is also increasing. This also presents significant challenges as technologists and attorneys continually work to learn how to process, search, and produce newer and increasingly complicated cloud-based data sources. The good news is that advanced analytics platforms can also help manage new data types in an efficient and cost-effective manner. Some newer AI-based analytics platforms can provide a holistic view of an organization’s entire legal data portfolio and identify broad trends and insights – inclusive of every variety of data present within it. These insights can help reduce cost and risk and sometimes enable organizations to upgrade their entire eDiscovery program. A holistic view of organizational data can also be helpful for outside counsel because it also enables better and more strategic legal decisions for individual matters and investigations.
3. The velocity, or the speed of data
Within eDiscovery, the velocity of data not only refers to the speed at which new data is generated, but also the speed at which data can be processed and analyzed. With smaller data volumes, it was manageable to put all collected data into a database and analyze it later. However, as data volumes increase, this method is expensive, time consuming, and may lead to errors and data gaps. Once again, a big data analytics product can help overcome this challenge because it is capable of rapidly processing and analyzing iterative volumes of collected data on an ongoing basis. By processing data into a big data analytics platform at the outset of a matter, counsel can quickly gain insights into that data, identifying relevant information and potential data gaps much earlier in the processes. In turn, this can mean lower data hosting costs as objectively non-responsive data can be jettisoned prior to data hosting. The ability of big data analytics platforms to support the velocity of data change also enables counsel and reviewers to be more agile and evolve alongside the constantly changing landscape of the discovery itself (e.g., changes in scope, custodians, responsive criteria, court deadlines).
4. The veracity, or uncertainty of data
Within the eDiscovery realm, the veracity of data refers to the quality of the data (i.e., whether the data that a party collects, processes, and produces is accurate and defensible and will satisfy a discovery request or subpoena). The veracity of the data produced to the opposing side in a litigation or investigation is therefore of the utmost importance, which is why data quality control steps are key at every discovery stage. At the preservation and collection stages, counsel must verify which custodians and data sources may have relevant information. Once that data is collected and processed, the data must then be checked again for accuracy to ensure that the collection and processing were performed correctly and there is no missing data. Then, as data is culled, reviewed, and prepared for production, multiple quality control steps must take place to ensure that the data slated to be produced is relevant to the discovery request and categorized correctly with all sensitive information appropriately identified and handled. As data volumes grow, ensuring the veracity of data only becomes more daunting.
Thankfully, big data analytics technology can also help safeguard the veracity of data. Cutting-edge AI technology can provide a big-picture view of an organization’s entire legal portfolio, enabling counsel to see which custodians and data sources contain data that is consistently produced as relevant (or, in the alternative, has never been produced as relevant) across all matters. It can also help identify missing data by providing counsel with a holistic view of what was collected in past matters from data sources. AI-based analytics tools can also help ensure data veracity on the review side within a single matter by identifying the inevitable inconsistencies that happen when humans review and categorize documents within large volumes of data (i.e., one reviewer may categorize a document differently than another reviewer who reviewed an identical or very similar document, leading to inconsistent work product). Newer analytics technology can more efficiently and accurately identify those inconsistencies during the review process so that they can be remedied early on before they cause problems.
Big Data Analytics-Based Methodologies
As shown above, AI-based big data analytics platforms can help counsel manage growing data volumes in eDiscovery.
For a more in-depth look at how a cutting-edge analytics platform and big data methodology can be applied to every step of the eDiscovery process in a real-world environment, please see Lighthouse’s white paper titled “The Challenge with Big Data.” And, if you are interested in this topic or would like to talk about big data and analytics, feel free to reach out to me at KSobylak@lighthouseglobal.com.
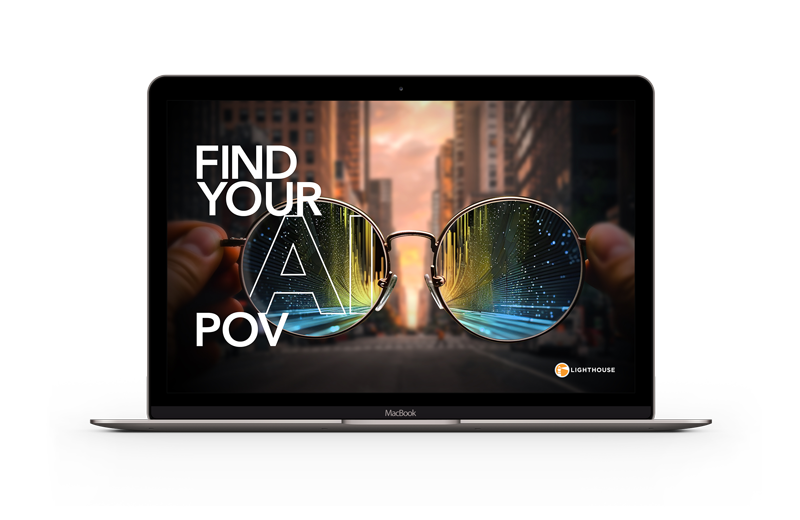