Analytics and Predictive Coding Technology for Corporate Attorneys: Demystifying the Jargon
August 5, 2021
By:
Below is a copy of a featured article written by Jennifer Swanton of Medtronic, Shannon Capone Kirk of Ropes & Gray, and John Del Piero of Lighthouse for Legaltech News.
Despite the traditional narrative that lawyers are hesitant to embrace technology, many in-house legal departments and their outside service providers are embracing the use of what is generally referred to as artificial intelligence (AI). In terms of litigation and internal investigations, this translates more specifically into conceptual analytics and predictive coding (also referred to as continuous active learning, or CAL), which are two of the more advanced technological innovations in the litigation space and corporate America.
This adoption, in part, seems to be driven by an expectation from corporate leaders that their in-house counsel must be able to identify and utilize the best available technology in order to drive cost efficiency, while also reducing risk and increasing effective and defensible litigation positions. For instance, in a 2019 survey of 163 legal professionals conducted by ALM Intelligence and LexisNexis, 92% of attorneys surveyed planned to increase their use of legal analytics in the upcoming 12 months. The reasoning behind that expected increase in adoption was two-fold, with lawyers indicating that it was driven both by competitive pressure to win cases (57%), as well as client expectation (56%).
Given that the above survey took place right before the COVID-19 pandemic hit, it stands to reason that the 92% of attorneys that expected to increase their use of analytics tools in 2020 may actually be even higher now. With a divisive election and receding pandemic only recently behind us, and an already unpredictable market, many corporations are tightening budgets and looking to further reduce unnecessary spend. Conceptual analytics and CAL are easy (yes, really) and effective ways to manage ballooning datasets and significantly reduce discovery, litigation and internal investigation costs.
With that in mind, we would like to help create a better relationship between corporate attorneys and advanced technology with the following two step approach—which we will outline in a series of two articles.
This first installment will help demystify the language technology providers tend to use around AI and analytics technology so that in-house teams feel more comfortable with adoption. In our second article, we will provide examples of some great use cases where corporate legal teams can easily leverage technology to help improve workflows. Together, we hope this approach can help in-house legal teams adopt technology that drives efficiency, lowers cost, and improves the quality of their work.
Demystifying AI Jargon
If you have ever discussed AI or analytics technology with a technology provider, you are probably more than aware that tech folks have a tendency to forget that the majority of their clients don’t live in the world of developing and evaluating new technology, day in and day out. Thus, they may use terms that are often confusing to their legal counterparts (and sometimes use terms that don’t match what the technology is capable of in the legal world). For this reason, it is helpful to level set with some common terminology and definitions, so that in-house attorneys are prepared to have better, more practical real-world discussions with technology providers.
Analytics Technology: Within the eDiscovery and compliance space, analytics technology is the ability of a machine to recognize patterns, structures, concepts, terminology, and/or the people interacting within data, and then present that analysis in a visual representation so that attorneys have a better overview of their data. As with AI, not all analytics tools have the same capabilities. Vendors may label everything from email threading identification to more advanced technology that can identify complex concepts and human sentiment as “analytics” tools.
Within these articles, when we reference this term, we are referring to the more advanced technology that can analyze not only the text within data but also the metadata and any previous coding applied by subject matter experts. This is an important distinction because this type of technology can greatly improve the accuracy of the analysis compared to older tools. For example, analytics technology that can analyze metadata as well as text is much better at identifying concepts like attorney-client privilege because it can analyze not only the language being used but who is using that language and the circumstances in which they use it.
Artificial Intelligence (AI): Probably the most broadly recognized term due to its prevalence outside of the eDiscovery space, AI is technically defined as the ability of a computer to complete tasks that usually would require human intelligence. Within the eDiscovery and compliance world, vendors often use the term broadly to refer to a variety of technologies that can perform tasks that previously would require completely human review.
It is important to remember though that the term AI can refer to a broad range of technology with very different capabilities. “AI” in the legal world is currently being used as a generalized term and legal consumers of such technologies should press for specifics—not all “AI” is the same, or, in several cases, even AI at all.
Machine Learning: Machine learning is a category of algorithms used in AI that can analyze statistics and find patterns in large volumes of data. The algorithms improve with experience—meaning that as documents are coded in a consistent fashion by humans, the better and more accurate the algorithms should become at identifying specific data types. Note here that there is a common misunderstanding that machine learning requires large amounts of data from which to learn. That is not necessarily true—all that is required for machine learning to work well is that the input it learns from (i.e., document coding for eDiscovery purposes) is consistent and accurate.
Natural Language Processing (NLP): NLP is a subset of AI that uses machine learning to process and analyze the natural language humans use within large amounts of data. The result is technology that can “understand” the contents of documents, including the context in which language is used within them. Within eDiscovery, NLP is used within more advanced forms of analytics technology to help identify specific content or sentiments within large datasets.
For example, NLP can be used to more accurately identify sensitive information, like personally identifiable information (PII), within datasets. NLP is better at this task than older AI technology because older models relied on “regular expressions” (a sequence of characters to define a search pattern) to identify information. When a “regular expression” (or regex) is used by an algorithm to find, for example, VISA account numbers—it will be able to identify the correct number pattern (i.e., any number that starts with the number 4 and has 16 digits) within a dataset but will be unable to differentiate other numbers that have the same pattern (for example, employee identification numbers). Thus, the results returned by legacy technology using regex may be overbroad and include false positives.
NLP can return more accurate results for that same task because it is able to identify not only the number pattern, but can also analyze the language used around the pattern. In this way, NLP will understand the context in which VISA account numbers are communicated within that dataset compared to how employee identification numbers are communicated, and only return the VISA numbers.
Predictive Coding (also referred to as Technology-Assisted Review or TAR): Predictive coding is not the same as conceptual analytics. Also, predictive coding is a bit of a misnomer, as the tools don’t predict or code anything. A human reviewer is very much involved. Simply put, it refers to a form of machine learning, wherein humans review documents and make binary coding calls: what is responsive and what is non-responsive. This is similar in concept to selecting thumbs up or down in Pandora so as to teach the app what songs you like and don’t like. After some human coding and calibrations between the human and the tool, the technology uses the human’s coding selections to score how the remaining documents should be coded, enabling the human to review the high scored documents first.
In the most current versions of predictive coding, this technology continually improves and refreshes as the human reviews, which reduces or eliminates the need for surgical precision on coding at the start (which was a concern in the former version of predictive coding and why providers and parties spent a considerable amount of time concerned with “seed sets”). This improved and self-improving prioritization of large document sets based on high-scored documents is usually a more efficient and organized manner in which to review documents.
Because of this evolution in predictive coding, it is often referred to in a host of different ways, such as TAR 1.0 (which requires “seed sets” to learn from at the start) and TAR 2.0 (which is able to continually refresh as the human codes—and is thus also referred to as Continuous Active Learning or CAL). Some providers continue to use the old terminology, or explain their advancements by walking through the differences between TAR 1.0 and TAR 2.0, and so on. But, speaking plainly, in this day and age, providers and legal teams should really only be concerned with the latest version of TAR, which utilizes CAL, and significantly reduces or totally eliminates the previous concern with surgical precision on coding an initial “seed set.” With our examples in the next installment, we hope to illustrate this point. In a word, walking through the technological evolution around predictive coding and all of the associated terminology can cause unnecessary intimidation, and can cause confusion between providers, parties and the court.
The key takeaway from these definitions is that even though all the technology described above may technically fall into the “AI” bucket, there is an important distinction between predictive coding/TAR technology and advanced analytics technology that uses AI and NLP. The distinction is that predictive coding/TAR is a much more technologically-limited method of ranking documents based on binary human decisions, while advanced analytics technology is capable of analyzing the context of human language used within documents to accurately identify a wide variety of concepts and sentiment within a dataset. Both tools still require a good amount of interaction with human reviewers and both are not mutually exclusive. In fact, on many investigations in particular, it is often very efficient to employ both conceptual analytics and TAR, simultaneously, in a review.
Please stay tuned for our next installment in this series, “Analytics and Predictive Coding Technology for Corporate Attorneys: Six Use Cases”, where we will outline six specific ways that corporate legal teams can put this type of technology to work in the eDiscovery and compliance space to improve cost, outcome, efficiencies.
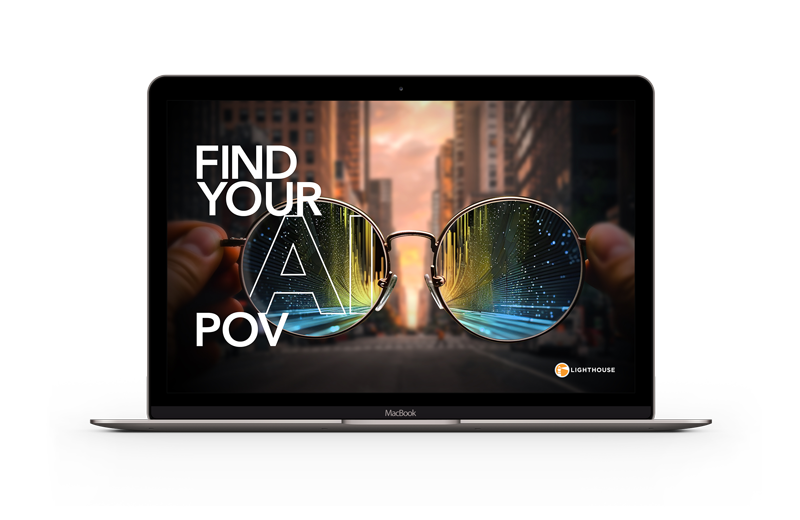