ESI Protocols in the Age of AI: 4 Essential Questions for Modern Discovery
May 13, 2025
By:
Summary: With the evolution of AI capabilities and adoption outpacing procedural frameworks, its important to reevaluate your approach to ESI protocols. Learn about four key considerations that will help you to create effective and defensible protocols that account for AI as both tool and data source.
When it comes to implementing AI in legal workflows and beyond, keeping up is often the name of the game. Changes to Electronically Stored Information (ESI) protocols are no different: They must increasingly account for AI tools that have become integral to discovery workflows. With AI approaches (including predictive, generative, and now agentic) evolving faster than procedural frameworks, thoughtful protocol development has never been more important for reckoning with data complexity, defensibility, and transparency in methodology.
New ESI tools, new ESI rules
ESI protocols, established in response to FRCP amendments, lay the mutually-agreed groundwork for how parties identify, review, and exchange information during litigation. When meet-and-confer is productive and well-negotiated, it creates a solid foundation for defensible discovery and has the added benefits of smoother workflows and fewer disputes. But with AI now an instrument of efficiency and scale, pointed at decision-making in document review—in fact, our State of AI in eDiscovery report found that 95% of respondents have medium to high trust in AI for eDiscovery uses—many traditional protocols have fallen behind current practices.
Generative AI prompts and outputs are ESI, too
While we account for the use of AI in analysis, review, and production, there’s a whole other class of AI usage that also produces potentially discoverable information with significance for internal investigations and disputes: productivity-based generative AI, including Microsoft Copilot, ChatGPT, and Google Gemini. Interactions with these tools produce not just prompts but responses. Some (like Copilot, due to its intra-enterprise deployment) have configurable storage and retention mechanisms for the data produced in the interactions; many others don’t store prompt and response history, or retain it only temporarily. And there’s the challenge of shadow IT—unauthorized use of AI for work—with its lack of visibility making upstream data handling difficult if not impossible.
Four questions for protocol development
To create effective, defensible ESI protocols that properly account for AI both as a tool and as a data source, consider these key questions during your planning process:
Question 1: Where and how does AI support discovery processes?
Start by mapping exactly where AI tools appear in your workflow:
- Are they helping prioritize documents for human review?
- Are they identifying privilege and creating privilege logs?
- How do they complement or replace traditional review approaches?
Different applications carry different levels of risk and require appropriate documentation in your protocol.
Question 2: What level of transparency makes sense for your matter?
FRCP Rule 26(b)(1) stresses proportionality, weighing the necessity of transparency in how AI models are trained and tuned versus the risks of IP exposure and the burden of producing this information. Consider this balance in relation to:
- Which AI tools will be used
- The level of process transparency reasonable to expose and/or be expected by the presiding judge
- Your approach to quality control documentation
The nuance of transparency can be especially challenging to juggle with confidentiality and relevance when precedent is still emerging.
Question 3: How will you demonstrate defensibility?
Decisions made or augmented by AI must be explicable. Part of protocol development should be to document how both parties expect defensibility to be proven, including:
- Explaining methodology in understandable terms
- Describing the validation process (sampling methods, testing)
- Outlining what human oversight measures were in place
Keep in mind, too, that defensibility looks different depending on the type of AI. Predictive AI relies on training sets and validation stats like precision and recall to support a clear path of reasoning. Generative AI defensibility is a bit more nebulous, as is the macro recommendation for deploying generative AI. Human oversight is crucial, as it’s the primary way to support what was reviewed and accepted.
Question 4: Does the matter type need to account for relevant generative AI prompts and outputs?
IP litigation against a former employee, for instance, might be more comprehensive with the preservation of their Microsoft Copilot data. Employment and contract disputes could also be well-served with negotiating this data source in protocol development.
Other questions to consider:
- Do internal legal holds explicitly cover AI tools?
- Is there enterprise logging or retention for AI interactions?
- How will prompts and responses be exported for review and produced—what will the format be?
Perfect is the enemy of good
Courts have highlighted the importance of flexibility in ESI protocols. In Morse Elec., Inc. v. Stearns, the court enforced the ESI protocol as controlling but noted that parties should seek timely judicial relief if compliance becomes challenging, emphasizing that protocols should not be "carved in stone." As courts increasingly expect transparency around AI use in discovery, legal teams must adapt their protocols accordingly—but fear of adherence to protocols shouldn’t be a limiting factor in whether to use AI (or TAR). By guiding protocol development with consideration of novel data sources, use cases, defensibility, and process transparency, you provide a solid framework for incorporating AI into eDiscovery.
To learn more about identifying and mitigating risks associated with AI and other new data types, visit our Strategic Consulting Services page.
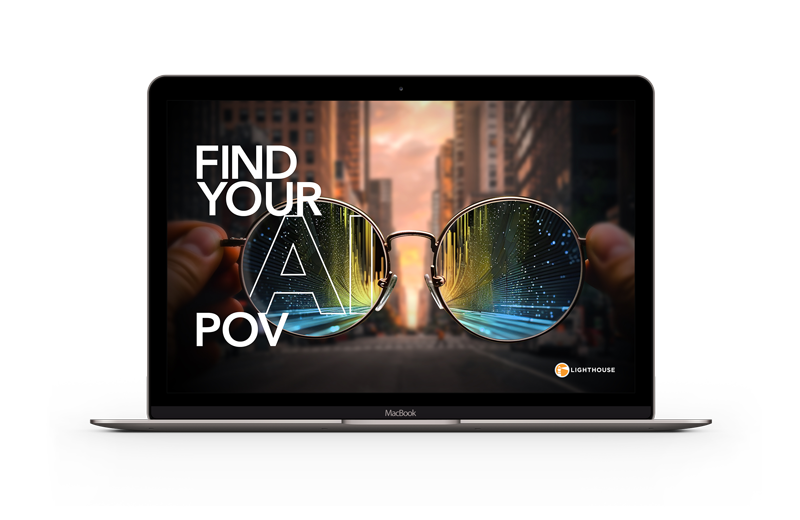