3 Reasons Traditional Document Review Isn’t Flexible Enough for Your Needs
August 16, 2023
By:
Modern data volumes and complexity have ushered in a new era of document review. The traditional approach, in which paid attorneys manually review all or most documents in a corpus, fails to meet the intense needs of legal teams today.
Specifically, legal teams need to:
• Scale their document review capability to cover massive datasets
• Rapidly build case strategy from key information hidden in those datasets
• Manage the risk inherent in having sensitive and regulated information dispersed across those datasets
Advanced review technology—including AI-powered search tools and analytics—enables teams to meet those needs, while simultaneously controlling costs and maintaining the highest standards of quality and defensibility. Rather than ceding decisions to a computer, reviewers are empowered to make faster decisions with fewer impediments. (For a breakdown of how technology sets reviewers up for success, see our recent blog post). In a nutshell, legal teams that use advanced technology can be more flexible, tackling large datasets with fewer resources, and addressing strategy and risk earlier in the process.
A flexible approach to scale: refining the responsive set
Responsiveness is the center of all matters. With datasets swelling to millions of documents, legal teams must reduce the responsive set defensibly, cost-efficiently, and in a way they can trust.
With traditional eyes-on review, the only way to attempt this is to put more people or hours on the job. And this approach requires reviewers to make every coding decision, which is often mentally taxing and prone to error.
Advanced review technology is purpose-built to scale for large datasets and provide a more nuanced assessment of responsiveness. Namely, it assigns a probability score—say, a given document is 90% or 45% likely to be responsive—that you can use to guide the review team. Often this means reviewers start with the highest-probability docs and then proceed through the rest, eventually making their way through the whole corpus.
But legal teams have a lot of flexibility beyond that. Combining machine learning with rules-based linguistic models can make responsive sets vastly more precise, decreasing both risk and downstream review costs.
Using this approach, machine learning is leveraged for what it does best—identifying clearly responsive and clearly non-responsive materials. For documents that fall in the middle of machine learning’s scoring band—those the model is least certain about—linguistic models built by experts target responsive language found in documents reviewed by humans, and then expand out to find documents with similar language markers. This approach allows legal teams to harness the strengths of both computational scalability and human reasoning to drive superior review outcomes.
A flexible approach to strategy: finding key documents faster
Only about 1% to 1.5% of a responsive set consists of key documents that are central to case planning and strategy. The earlier legal teams get their hands on those documents, the sooner they can start on that invaluable work.
Whereas it takes months to find key documents with traditional review, advanced technology shortens the process to mere weeks. This is because key document identification utilizes complex search strings that include key language in context. For example, “Find documents with phrase A, in the vicinity of phrases B, C, and D, but not in documents that have attributes E and F,” and so on.
A small team of linguistic experts drafts these searches and refines them as they go, based on feedback from counsel. In one recent matter, this approach to key document identification proved 8 times faster than manual review, and more than 90% of the documents it identified had been missed or discarded by the manual review team.
The speed and iterative nature of this process are what enable legal teams to be more flexible with case strategy. First, they have more time to choose and change course. Second, they can guide the search team as their strategy evolves, ensuring they end up with exactly the documents they need to make the strongest case.
A flexible approach to risk: assessing privilege and PII sooner and more cost effectively
Reviewing for privilege is a notoriously slow and expensive part of eDiscovery. When following a traditional approach, you can’t even start this chore until after the responsive set is established.
With advanced technology, you can review for privilege, PII, and other classifications at the same time that the responsive set is being built. This shortens your overall timeframe and gives you more flexibility to prepare for litigation.
Legal teams can even be flexible with their privilege review budget. As with responsiveness, advanced technology will rate how likely a document is to be privileged. Legal teams can choose to send extremely high- and low-scoring documents to less-expensive review teams, since those documents have the least ambiguity. Documents that score in the middle have the most ambiguity, so they can be reviewed by premium reviewers.
It’s all about options in the end
Broadly speaking, the main benefit of supporting document review with advanced technology is that it gives you a choice. Legal teams have the option to start key tasks sooner, calibrate the amount and level of eyes-on review, and strategize how they use their review budgets. With linear review, those options aren’t available.
Legal teams that give themselves these options, by taking advantage of supportive technology, are better able to scale, strategize, and manage risk in the modern era of document review.
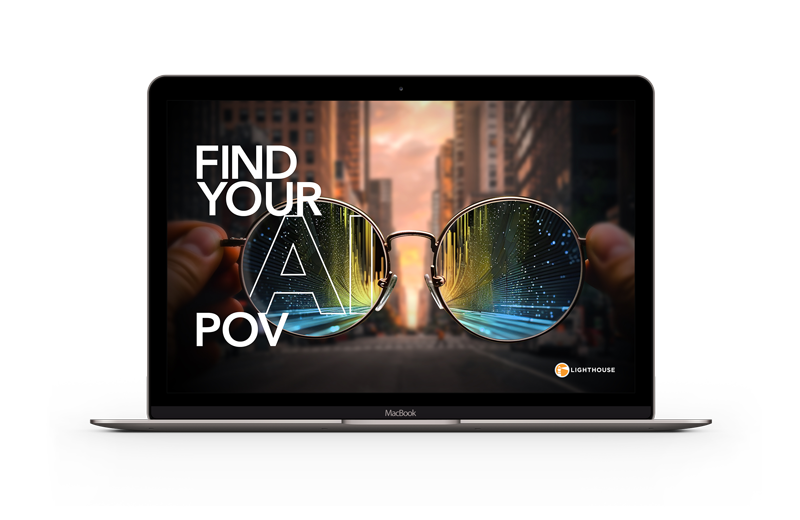